The role of radiomics in hepato-bilio-pancreatic surgery: a literature review
Abstract
Radiomics is an advanced computational analysis of biomedical images that aims to obtain a detailed, objective, and multidimensional characterization of biological tissues. Radiomics features ultimately represent the physiopathology of the tissue under study and can be used to characterize and quantify the spatial distribution and interactions between the voxels that compose a biomedical image. The aim of this paper was to review the current role of radiomics in hepato-bilio-pancreatic surgery by analyzing systematic reviews, meta-analyses and the most relevant published series. Literature data revealed that radiomics is a promising tool in improving the non-invasive characterization and preoperative staging of hepato-bilio-pancreatic neoplasms. Nevertheless, there are major limitations in this approach, mainly linked to the lack of standardization in image acquisition, that result in a significant translational gap between research and clinical practice.
Keywords
INTRODUCTION
Hepato-bilio-pancreatic (HBP) surgery comprises demanding and complex procedures, the planning of which requires highly specific radiological skills for diagnosis, staging, and evaluation of treatment response. The interpretation of imaging results, in view of the clinical findings, relies heavily on the training and experience of the radiologist; as such, the conventional process of image interpretation has limited diagnostic accuracy and reproducibility. Moreover, radiological images contain a large amount of information that is invisible even to an experienced human eye.
Radiomics is an advanced computational analysis of biomedical images, including ultrasound (US), computed tomography (CT), magnetic resonance imaging (MRI), and positron emission tomography (PET), that aims to obtain an objective, detailed, and multidimensional characterization of biological tissues through the extraction of numerical features by converting images into mineable data. Radiomics features numerically describe and quantify the spatial distribution and relationships between the voxels that compose a “black and white” image, which ultimately reflect the underlying physiopathology of the tissue under study[1]. Although the clinical role of radiologists in patients’ care remains a cornerstone of cancer imaging, the addition of radiomics features to the visual assessment of an imaging examination may improve the diagnostic performance of radiologists, the reproducibility of the results, and the patients’ outcomes by identifying adverse pathological features, predicting disease recurrence and survival, and improving the evaluation of treatment response, which are extremely important for HBP surgery. Previous reports revealed that radiomics is a promising tool to improve the non-invasive characterization and preoperative staging of HBP neoplasms[1]. Nevertheless, each individual step in the process of radiomics has technical challenges that result in a significant translational gap between research and clinical practice.
The aim of this paper was to review the current role of radiomics in HBP surgery by analyzing systematic reviews, meta-analyses and the most relevant published series.
RADIOMICS: WORKFLOW, POTENTIAL AND LIMITATIONS
The most important field of application of radiomics is oncological imaging. The underlying hypothesis is that radiomics features parallelize the heterogeneity that characterizes tumor histology, allowing deep exploration of tumor microenvironment and intra- and inter-tumoral heterogeneity, which are ultimately related to the biological and genomic characteristics[2]. The workflow of radiomics consists of several steps[3]: (1) acquisition of standardized, high-quality radiological images; (2) accurate segmentation of the tumor mass with delineation of a volume of interest (VOI); (3) extraction of reproducible, non-redundant and uncorrelated radiomics features; (4) integration of radiomics features with pathological and clinical data; (5) construction of a database for data mining.
Each of these steps is a potential source of bias that may affect the quality, robustness and reproducibility of the results. Given that a single voxel can influence the radiomics features, differences in the equipment, for example, the magnetic field strength in MRI and the number of detectors in CT, and the image acquisition protocols lead to relevant discrepancies between studies.
Segmentation, defined as the delineation of the tumor mass relative to adjacent structures, is one of the most important sources of variability. Manual tumor segmentation is time-consuming and limited by human capabilities, but also has the advantage of being controlled in real time by the human eye; on the other hand, automatic tumor segmentation is fast and highly standardizable, but lacks the ability to iteratively understand whether data are being acquired correctly; finally, semi-automatic tumor segmentation seems to be the most effective method because it combines the advantages of computer technology with the control of the human eye[3]. Radiomic feature extraction is a poorly standardized process, given the multitude of software applications that work differently to convert voxels to numerical data; furthermore, post-processing of biomedical images can be done with several different modeling algorithms. Both these aspects increase the heterogeneity between studies. Finally, large, shared databases for data mining would be essential to validate the results of single studies by interrogating separate populations of patients and to obtain study cohorts with sufficient size for statistical power.
Radiomic research initially used manual tumor segmentation and conventional statistical tests, such as the Fisher’s or Wilcoxon tests, to select radiomics features; more complex statistical analyses, such as the least absolute shrinkage and selection operator (LASSO), were then used to select predictive features. Although this approach is easy to perform even by operators without specific experience in image processing and statistical analysis, it is time-consuming and its application is limited to small populations. The current research trend relies on machine learning (ML) methods, as they allow for a higher level of automation compared to the traditional workflow, providing faster segmentation and feature extraction as well as advantages in terms of reproducibility. Despite these benefits, ML-based radiomics are limited by the high correlation to the quality of the input data (i.e., the accuracy of the segmentation and the size of the training population); therefore, large datasets are necessary to identify robust features.
Researchers’ interest in radiomics has exploded in recent years, and thousands of studies on several different settings have been published. As a decade of radiomics research is approaching, it is time for a critical review of what results have been achieved and what has been translated into clinical practice. The Radiomics Quality Score (RQS) was developed to evaluate the methodological quality of a radiomic study[4]. The RQS consists of 16 criteria, with a score of 36 (or 100%) indicating excellent study quality [Table 1]. A recent study[5] reported a median RQS of 21% among 44 systematic reviews on radiomics, suggesting that the quality in this research field needs to be increased as it is currently unsatisfactory regardless of the topic.
Items composing the radiomics quality score and relative points[4]
RQS checkpoints | Criteria | Points |
1 | Image protocol quality | +1 or +2 |
2 | Multiple segmentation | +1 |
Phantom study | +1 | |
Imaging at multiple timepoints | +1 | |
3 | Feature reduction or adjustment for multiple testing | -3 or +3 |
Multivariable analysis | +1 | |
Biological correlates | +1 | |
Cut-off analysis | +1 | |
Discrimination statistics | +1 or +2 | |
Calibration statistics | +1 or +2 | |
Prospective study | +7 | |
Validation | -5 to +5 | |
Comparison to gold standard | +2 | |
Potential clinical applications | +2 | |
Cost-effectiveness analysis | +1 | |
Open science and data | +1 to +4 |
METHODS
A search of the MEDLINE database was performed to identify meta-analysis and systematic reviews relevant to detection, characterization and differential diagnosis, identification of adverse pathological features, and prediction of prognosis of hepato-bilio-pancreatic neoplasms using radiomics. The following terms were searched: “(liver OR hepatic) AND (radiomics OR histogram analysis)”, “pancreas AND (radiomics OR histogram analysis)”, “(hepatocellular carcinoma OR hepatocarcinoma) AND (radiomics OR histogram analysis)”, “cholangiocarcinoma AND (radiomics OR histogram analysis)”, “liver metastases AND (radiomics OR histogram analysis)”, “pancreatic adenocarcinoma AND (radiomics OR histogram analysis)”, “pancreatic neuroendocrine AND (radiomics OR histogram analysis)”, “intraductal papillary mucinous neoplasm AND (radiomics OR histogram analysis)”. A total of 38 meta-analyses and systematic reviews were retrieved; the search was further expanded by reviewing the reference list of the selected articles in order to identify relevant papers in terms of sample size, originality, methodology and importance of the results. Full-text articles in English, reporting on US, CT, and MRI, published by 15th May 2023, were considered. Studies were excluded if they were not conducted in humans or if they evaluated other imaging techniques such as PET-CT or endoscopic US. Seventy-one studies met the eligibility criteria and were included in this literature review. The results of the meta-analysis and systematic reviews that reported pooled data diagnostic values are summarized in Table 2; the studies analyzed are described in the following paragraphs.
Summary of the meta-analysis and systematic reviews included in this study that reported pooled diagnostic values
Study | Aims | Diagnostic value |
Harding-Theobald et al.[10] | Differentiation of HCC from other lesions Prediction of MVI in HCC Prediction of recurrence after hepatectomy for HCC Prediction of prognosis after treatment for HCC | c-statistic 0.66-0.95 c-statistic 0.76-0.92 c-statistic 0.71-0.86 c-statistic 0.74-0.81 |
Huang et al.[17] | Preoperative prediction of MVI in HCC | Se 0.78, Sp 0.78 |
Wang et al.[18] | Preoperative prediction of MVI in HCC | AUC 0.69-0.94 |
Li et al.[19] | Preoperative prediction of MVI in HCC | Se 84%, Sp 83%, AUC 0.90 |
Zhong et al.[20] | Preoperative prediction of MVI in HCC | AUC 0.74-0.87 |
Fiz et al.[31] | Lymph node metastases in biliary tumors Grading in biliary tumors Survival in biliary tumors Differentiation of iCC from other lesions | AUC 0.729-0.900, Acc 0.69-0.83 AUC 0.680-0.890, Acc 0.70-0.82 C-index 0.673-0.889 AUC > 0.800 |
Wesdorp et al.[44] | Response to treatment in LM | AUC 0.797-0.814 |
Jia et al.[45] | Preoperative prediction of KRAS status in LM | Se 0.80/0.78, Sp 0.80/0.84, AUC 0.87/0.86 |
Gao et al.[52] | Correlation with OS in PDAC | HR 1.66 |
Staal et al.[53] | Prediction of tumor grade in GEP-NETs Differentiation of GEP-NETs from other lesions Recurrence in pNETs | AUC 0.74-0.96 AUC 0.80-0.99 AUC 0.77 |
HEPATOCELLULAR CARCINOMA
Hepatocellular carcinoma (HCC) is usually diagnosed and monitored by imaging; therefore, it is a good candidate for radiomic analysis. Several studies have been published regarding the identification, diagnosis, and prediction of adverse pathological features, and prognosis in HCC patients.
Diagnosis
Transabdominal US, alone or in combination with serum markers, is the backbone for surveillance and early identification of HCC in high-risk subjects[6,7], as it has a sensitivity of 94% for detecting HCC before it becomes clinically apparent; however, US has a lower sensitivity (63%) for detecting early-stage HCC[8]. There are several reasons for this, including tumor location, size, and echogenicity, as well as patient-related factors that limit US exploration, such as poor cooperation, obesity, and marked steatosis, which are relevant given the increasing prevalence of non-alcoholic fatty liver disease (NAFLD). Enhancing the diagnostic capability of US for early identification of HCC would be highly beneficial from a clinical point of view. Radiomics has a potential role in this regard, although the evidence is very scarce: one single study by Yao et al. explored radiomic analysis in 177 subjects with a liver lesion who underwent B-mode US, shear wave elastography, and shear wave viscosity imaging; 2,560 features were extracted and five radiomic models were constructed to differentiate between benign and malignant lesions and to diagnose HCC[9]. The areas under the curve (AUCs) were 0.94 for differentiation between benign and malignant lesions and 0.97 for classification of the malignant subtype. Although CT and MR are frequently performed after an initial insufficient US examination of the liver, their role in screening has not been investigated, as both are unsuitable due to radiation burden and high cost, respectively. Nine of the 54 studies included in the systematic review by Harding-Theobald et al. examined aspects of HCC diagnosis based on radiomics analysis, but most of them focused on the differentiation between hemangiomas from HCC, which is a simple task for trained Radiologists; moreover, these studies were of low methodological quality, with a RQS ranging from 5 to 10[10]. Dankerl et al. trained a computer-aided diagnosis (CAD) system based on CT texture analysis in 372 patients with 2,325 liver lesions[11]. The diagnostic performance of the CAD system for differentiation between benign and malignant lesions and between cysts, hemangiomas, and metastases was high, with AUCs of 94.5% for lesion type and 91.4% for lesion histology; overall, the CAD system performed better than three radiologists blinded to clinical information and with access only to CT images. Using the European Association for the Study of the Liver (EASL) guidelines, Mokrane et al. retrospectively examined 178 patients with cirrhosis with radiologically indeterminate, biopsy-proven liver nodules[12]. 142 and 36 patients were randomly chosen for discovery and validation cohorts, respectively. Nodules were segmented on CT images, and 13,920 features were extracted. The radiomic signature was trained, calibrated, and validated using machine learning for differentiation between HCC and non-HCC nodules: a single radiomics feature had an AUC of 0.70 and 0.66 in the two cohorts. Laino et al. reviewed 11 studies on automatic classification of liver nodules using the Liver Imaging Reporting and Data System (LI-RADS) criteria: all studies demonstrated that radiomics performs well in the classification of liver nodules, sometimes better than human evaluation, reaching an AUC of 0.98 either on CT or MR[13].
Prognostic stratification
Prognostic stratification is the most challenging and fascinating application of radiomics. This can be obtained either by correlating radiomics features to known adverse pathological features or by directly comparing radiomics features to overall survival (OS) and recurrence-free survival (RFS). Tumor grade has a role in determining the prognosis of HCC patients, as disease recurrence and metastasis are more likely to develop in high-grade HCCs[14]. Mao et al.[15] and Wu et al.[16] reported high AUC values for combined clinical and radiomics models for HCC grading prediction (0.801 and 0.800, respectively). Microvascular invasion (MVI) has an impact on survival outcomes and disease recurrence. Studies conducted on the role of radiomics in predicting MVI reported AUC values ranging from 0.76 to 0.94[10,17-20], with MR-based studies having the highest diagnostic value in predicting MVI. Another negative prognostic factor in HCC is vessels encapsulated tumor clusters (VETC), which are histologically defined as the presence of vessels marked with CD34 completely encapsulating neoplastic clusters. Yu et al. developed a predictive model based on contrast-enhanced MR images obtained after gadolinium ethoxy benzylic diethylenetriamine pentaacetic acid (Gd-EOB-DTPA) injection, with AUC of 0.972 for preoperative prediction of VETC[21]. Finally, HCCs expressing biliary-specific markers such as CK19 have a higher rate of nodal metastasis and worse prognosis after surgery[22]. Wang et al. developed a radiomics-based model derived from MR images with sensitivity and specificity values of 0.818 and 0.974 in the training cohort and 0.769 and 0.818 in the validation cohort[23]. Disease recurrence after surgery negatively affects patients’ prognosis. According to 17 studies included in the meta-analysis by Harding-Theobald et al., radiomic models can predict early recurrence after surgery, with AUCs ranging from 0.71 to 0.86[10]. The radiomics model developed by Ji et al. to predict early disease recurrence outperformed non-radiomics models and commonly used staging systems in terms of predictive performance (C-index ≥ 0.77) and prediction error (integrated Brier
Response to treatment
Several radiological criteria have been proposed to assess treatment response in HCC patients, such as Response Evaluation Criteria In Solid Tumors (RECIST) and modified RECIST (mRECIST). Radiomics might enable a standardized, early and comprehensive evaluation of treatment response, with implications for patient management. Most studies were applied to patients who underwent loco-regional treatments for HCC[10]. Kim et al. demonstrated that post-TACE OS could be predicted with a hazard ratio (HR) of 19.8 by combining clinical (Child-Pugh score, alphafetoprotein, and tumor size) and radiomics features (surface area-to-volume ratio, kurtosis, median, size zone variability)[26]. Other studies reported similar results, indicating that radiomics features extracted from pre-treatment imaging were able to predict treatment response after TACE[27]. Histogram analysis of apparent diffusion coefficient (ADC) maps seems to predict TACE response, as reported by Wu et al.[28] and Shaghaghi et al.[29]. Yang et al.[30] developed a radiomics score composed of four features that independently affected recurrence after ablation at 1, 2, and 3 years with AUCs of 0.79/0.72, 0.72/0.61, and 0.71/0.64 in the train and validation groups, respectively.
CHOLANGIOCARCINOMA
Radiomics may help in diagnosis and treatment of biliary tumors. Fiz et al. included in their meta-analysis 27 studies with more than 3,600 patients[31]. Most studies focused on mass-forming intrahepatic cholangiocarcinoma (iCC). Radiomics predicted nodal metastases (AUC = 0.73-0.90, accuracy = 0.69-0.83), tumor grade (AUC = 0.68-0.89, accuracy = 0.70-0.82), and survival (C-index = 0.67-0.89); moreover, radiomics features allowed differentiation of iCC from HCC, combined HCC-iCC, and inflammatory lesions (AUC = 0.80). Differentiation of iCC from combined forms and atypical HCC may be difficult at imaging, but differentiation is necessary to enable optimal treatment decisions.
Diagnosis
Liu et al. aimed to differentiate combined HCC-CC from iCC and HCC using MRI and CT radiomics features[32]. MRI-based radiomics features were the best for differentiation of HCC-CC from non-HCC-CC (AUC = 0.77). Zhou et al. aimed to differentiate HCC-CC from mass-forming iCC by extracting radiomics features from contrast-enhanced MR images: a radiomics nomogram including alpha-fetoprotein, coexistent liver disease, and radiomics signature had an AUC value of 0.945 in the training cohort and 0.897 in the validation cohort[33].
Staging
Even using high-end preoperative imaging, understaging is a problem, as a substantial proportion of patients with cholangiocarcinoma (CC) have occult metastases detected only on resection specimens or through early recurrence after resection. Moreover, positive resection margins are not uncommon. For these reasons, preoperative risk stratification should be as precise as possible to better stratify patients’ prognoses. Ji et al. developed a radiomics signature with significant association with nodal metastases in a cohort of 155 patients (test cohort = 103 patients; validation cohort = 52 patients); by adding CA 19.9 levels to the radiomics signature, the model had AUC of 0.846 and 0.892 in the two cohorts, respectively[34]. Chu
Prediction of prognosis
Some studies have been published concerning the possibility of predicting the prognosis of patients with CC. The largest series in this regard was reported by a multicenter, retrospective study by Park et al., including 345 patients with mass-forming iCC who underwent surgery[40]. A clinical-radiologic model including infiltrative margins, multifocality, periductal infiltration, extrahepatic infiltration, and nodal metastases had similar performance in predicting RFS compared to the radiomics model (C-index, 0.65 vs. 0.68; P = 0.43). A clinical-radiological-radiomics model performed better than the clinical-radiologic model (C-index, 0.71; P = 0.01), with similar performance to commonly used postoperative prognostic systems to predict RFS (C-index, 0.71-0.73 vs. 0.70-0.73; P > 0.05) and OS (C-index, 0.68-0.71 vs. 0.64-0.74; P > 0.05). Zhang et al. developed an MRI-based texture signature predictive for the immunophenotyping and OS of patients with iCC: 78 patients were divided into two cohorts (inflamed iCC, n = 26; non-inflamed iCC,
Treatment selection and response to treatment
The radiomic feature Wavelet-HLH_firstorder_Median was associated with OS, with a C-index of 0.70. CT texture analysis can quantify vascularization and homogeneity of iCC, providing useful information in identifying optimal candidates for trans-arterial radioembolization (TARE), as reported by Mosconi et al.: in this study, analysis was retrospectively performed in 55 pre-TARE CT scans; iCCs showing objective response after TARE had a higher mean histogram values (P < 0.001), GLCM homogeneity (P = 0.005) and GLCM correlation (P = 0.030), and lower kurtosis (P = 0.043), Grey-level co-occurrence matrix (GLCM) contrast (P = 0.004), and GLCM dissimilarity (P = 0.005) at the pre-TARE CT scan[42].
LIVER METASTASES
Radiomics is a promising method to predict disease recurrence and survival and improve personalized treatment in patients with liver metastases (LM), according to three systematic reviews[43-45]. A very important issue was reported by Kelahan et al., as inter-reader reproducibility of CT radiomics features demonstrated tumor-size dependence, and this could explain result variability among the studies[46].
Prediction of Kirsten Rat Sarcoma Virus gene (KRAS) status
Kirsten Rat Sarcoma Virus gene (KRAS) mutation is associated with worse prognosis; on the other hand, KRAS inhibitors are more and more commonly used for the treatment of metastatic colorectal cancer. Predicting KRAS status with non-invasive methods would be, therefore, clinically useful. A meta-analysis by Jia et al. reported on the prediction of the KRAS status in patients with colorectal LM, with pooled sensitivity, specificity and AUC values of 0.80/0.78, 0.80/0.84 and 0.87/0.86 in the training and validation cohorts, respectively[45].
Response to treatment
Early and accurate response evaluation in patients with LM would be of importance given the availability of several different treatment modalities, and several studies[47-50] reported a significant association between radiomics features and response to chemotherapy and targeted therapies.
PANCREATIC NEOPLASMS
The potential usefulness of radiomics in preoperative staging and prediction of histological findings and clinical outcomes was reported by three systematic reviews and meta-analyses; these studies highlighted the low quality of most radiomics studies conducted so far. Abunahel et al. included 72 studies encompassing 8,863 participants; 66 studies investigated focal pancreatic lesions[51]. Overall, second-order features were the most useful for lesion characterization, while filtered image features were most useful for classification and prognosis predictions. The median RQS of studies included was 28%, and it was significantly correlated both with the amount of features (r = 0.52, P < 0.001) and the size of the study population (r = 0.34,
Early detection of pancreatic cancer
Despite improvements in the multidisciplinary treatment approach to patients with pancreatic ductal adenocarcinoma (PDAC), the prognosis of this disease remains very poor, as PDAC is frequently diagnosed at an advanced stage. The goal of screening and surveillance programs is to detect and treat stage I PDAC and cancer precursor lesions as intraductal papillary mucinous neoplasms (IPMNs) with high-grade dysplasia[54]. Even though encouraging results were described by two studies[55,56], a meta-analysis by Chhoda et al. reported a significant proportion of PDACs diagnosed at a late stage during follow-up, which limits the survival benefit of surveillance[57]. Although no studies have been conducted in this regard, AI and radiomics could theoretically play a role, as they can automatically differentiate between cancer and normal pancreas, as reported by Chu et al.: in this study, 427 features were extracted from CT images; overall, the accuracy of the binary random forest classification was 99.2%, with an AUC of 99.9%, a sensitivity of 100% and a specificity of 98.5%; a major limitation of this study is that the mean tumor size was 4.1 cm, which usually corresponds to a non resectable disease[58]. Two studies by Qureshi et al.[59] and Javed et al.[60] identified several features that may be predictive of PDAC development extracted from pre-diagnostic CT scans in PDAC patients: the predictive model had an accuracy of 86%-89.3%, a sensitivity of 86% and a specificity of 93%; unfortunately, these results were based on a very limited study population and needed further validation. The most robust study on early automated detection of PDAC was published by Mukherjee et al.: they used a radiomics-based machine learning model to detect PDAC before the clinical diagnosis based on volumetric segmentation of the pancreas performed on pre-diagnostic CT scans in 155 PDAC patients and 265 normal subjects[61]. A supporting vector machine model had high sensitivity (95.5%), specificity (90.3%), AUC (0.98), and accuracy (92.2%) for the classification of CT into pre-diagnostic versus normal. The paradigm for early detection of cancer in IPMN is based on “worrisome features” and “high-risk stigmata”. Accurate prediction of the malignant potential of IPMN is of great importance. Nevertheless, studies on AI/radiomic applications in identifying “malignant” IPMNs comprised very small study populations. The largest were proposed by Jeon et al.[62] and Chakraborty et al.[63], who reported that radiomics features improve the performance of MR and CT for predicting malignant IPMNs. Circulating micro RNAs (miRNAs) may be diagnostic biomarkers of incidentally detected IPMNs and predictors of their histological classification: a study by Permuth et al. suggested that the combination of radiomics features with the miRNA genomic classifier (MGC) had an AUC = 0.92 and sensitivity (83%), specificity (89%), PPV (88%), and NPV (85%) for prediction of IPMN malignancy[64].
Differential diagnosis
Radiomics is a promising tool to improve the characterization of focal pancreatic lesions. Zhang et al. developed CT and radiomics nomograms for the differentiation between mass-forming pancreatitis (MFP) and PDAC in patients with chronic pancreatitis (CP)[65]. 138 patients with histopathologically diagnosed MFCP or PDAC were retrospectively analyzed. Both models had good performance in differentiating between the two entities in the training (AUC = 0.87/0.91) and validation (AUC = 0.94) cohorts. A radiomics-based computer-aided diagnosis scheme proposed by Wei et al. had AUC = 0.767, sensitivity = 0.686, and
Prediction of prognosis
Tumor grade is a major prognostic factor for pancreatic neoplasms. Chang et al.[67] and Tikhonova et al.[68] reported significant differences in radiomics signatures between PDAC of different grades, with AUC ranging from 0.66 to 0.77. Tumor grade is even more important from a prognostic point of view for pNENs. Gu et al. included 138 patients with pathologically confirmed pNENs (training cohort, 104 patients; validation cohort, 34 patients)[69]. A nomogram integrating tumor margin status and a radiomic signature derived from CT images showed strong discrimination with AUCs of 0.974 and 0.902 in the training and validation cohort, respectively.
Several studies conducted a radiomic analysis to predict features with a negative prognostic role of pNENs. In particular, a study[70] retrospectively analyzed the MR histogram features of 42 patients with pNEN: ADCentropy was higher in G2-3 tumors with AUC = 0.757, sensitivity and specificity of 83.3% and 61.1%, while kurtosis was higher in pNENs with vascular infiltration, lymph node and liver metastases
CONCLUSION
Radiomics is a promising tool to improve the non-invasive characterization and the preoperative staging of HBP neoplasms, while the results concerning the prediction of patients’ clinical outcomes are still limited. Despite these promising results, radiomics is a young discipline and its application is still at the stage of research. There are several reasons for that, including technical complexity in image analysis, issues in study design, and lack of standards for image acquisition and validating results. Technical issues in image analysis and data extraction can be addressed easily through the collaboration between radiologists, computer scientists and physicists. A possible solution to poor reproducibility would be to establish precise benchmarks for radiomics studies, including acquisition protocols, and to develop guidelines for results reporting. Finally, data sharing is a relevant issue, as it includes images and a significant amount of personal information. A possible solution to overcome cultural, administrative, and regulatory issues is the creation of centralized data repositories with anonymized data, wherein access can be limited to institutional review board-approved users.
DECLARATIONS
Authors’ contributionsMade substantial contributions to the conception and design of the study and performed data analysis and interpretation: De Robertis R, Todesco M
Performed data acquisition: Autelitano D, Spoto F
Scientific guarantor of the study: D’Onofrio M
Availability of data and materialsNot applicable.
Financial support and sponsorshipNone.
Conflicts of interestAll authors declared that there are no conflicts of interest.
Ethical approval and consent to participateNot applicable.
Consent for publicationNot applicable.
Copyright© The Author(s) 2023.
REFERENCES
1. Gillies RJ, Kinahan PE, Hricak H. Radiomics: images are more than pictures, they are data. Radiology 2016;278:563-77.
2. Henry T, Sun R, Lerousseau M, et al. Investigation of radiomics based intra-patient inter-tumor heterogeneity and the impact of tumor subsampling strategies. Sci Rep 2022;12:17244.
3. Varghese BA, Cen SY, Hwang DH, Duddalwar VA. Texture analysis of imaging: what radiologists need to know. AJR Am J Roentgenol 2019;212:520-8.
4. Lambin P, Leijenaar RTH, Deist TM, et al. Radiomics: the bridge between medical imaging and personalized medicine. Nat Rev Clin Oncol 2017;14:749-62.
5. Spadarella G, Stanzione A, Akinci D’Antonoli T, et al. Systematic review of the radiomics quality score applications: an EuSoMII radiomics auditing group initiative. Eur Radiol 2023;33:1884-94.
6. Marrero JA, Kulik LM, Sirlin CB, et al. Diagnosis, staging, and management of hepatocellular carcinoma: 2018 practice guidance by the American Association for the study of liver diseases. Hepatology 2018;68:723-50.
7. European Association for Study of Liver, European Organisation for Research and Treatment of Cancer. EASL-EORTC clinical practice guidelines: management of hepatocellular carcinoma. Eur J Cancer 2012;48:599-641.
8. Singal A, Volk ML, Waljee A, et al. Meta-analysis: surveillance with ultrasound for early-stage hepatocellular carcinoma in patients with cirrhosis. Aliment Pharmacol Ther 2009;30:37-47.
9. Yao Z, Dong Y, Wu G, et al. Preoperative diagnosis and prediction of hepatocellular carcinoma: radiomics analysis based on multi-modal ultrasound images. BMC Cancer 2018;18:1089.
10. Harding-Theobald E, Louissaint J, Maraj B, et al. Systematic review: radiomics for the diagnosis and prognosis of hepatocellular carcinoma. Aliment Pharmacol Ther 2021;54:890-901.
11. Dankerl P, Cavallaro A, Tsymbal A, et al. A retrieval-based computer-aided diagnosis system for the characterization of liver lesions in CT scans. Acad Radiol 2013;20:1526-34.
12. Mokrane FZ, Lu L, Vavasseur A, et al. Radiomics machine-learning signature for diagnosis of hepatocellular carcinoma in cirrhotic patients with indeterminate liver nodules. Eur Radiol 2020;30:558-70.
13. Laino ME, Viganò L, Ammirabile A, et al. The added value of artificial intelligence to LI-RADS categorization: a systematic review. Eur J Radiol 2022;150:110251.
14. Zhou L, Rui JA, Wang SB, Chen SG, Qu Q. Clinicopathological predictors of poor survival and recurrence after curative resection in hepatocellular carcinoma without portal vein tumor thrombosis. Pathol Oncol Res 2015;21:131-8.
15. Mao B, Zhang L, Ning P, et al. Preoperative prediction for pathological grade of hepatocellular carcinoma via machine learning-based radiomics. Eur Radiol 2020;30:6924-32.
16. Wu M, Tan H, Gao F, et al. Predicting the grade of hepatocellular carcinoma based on non-contrast-enhanced MRI radiomics signature. Eur Radiol 2019;29:2802-11.
17. Huang J, Tian W, Zhang L, et al. Preoperative prediction power of imaging methods for microvascular invasion in hepatocellular carcinoma: a systemic review and meta-analysis. Front Oncol 2020;10:887.
18. Wang Q, Li C, Zhang J, et al. Radiomics models for predicting microvascular invasion in hepatocellular carcinoma: a systematic review and radiomics quality score assessment. Cancers 2021;13:5864.
19. Li L, Wu C, Huang Y, Chen J, Ye D, Su Z. Radiomics for the preoperative evaluation of microvascular invasion in hepatocellular carcinoma: a meta-analysis. Front Oncol 2022;12:831996.
20. Zhong X, Long H, Su L, et al. Radiomics models for preoperative prediction of microvascular invasion in hepatocellular carcinoma: a systematic review and meta-analysis. Abdom Radiol 2022;47:2071-88.
21. Yu Y, Fan Y, Wang X, et al. Gd-EOB-DTPA-enhanced MRI radiomics to predict vessels encapsulating tumor clusters (VETC) and patient prognosis in hepatocellular carcinoma. Eur Radiol 2022;32:959-70.
22. Lee CW, Kuo WL, Yu MC, et al. The expression of cytokeratin 19 in lymph nodes was a poor prognostic factor for hepatocellular carcinoma after hepatic resection. World J Surg Oncol 2013;11:136.
23. Wang W, Gu D, Wei J, et al. A radiomics-based biomarker for cytokeratin 19 status of hepatocellular carcinoma with gadoxetic acid-enhanced MRI. Eur Radiol 2020;30:3004-14.
24. Ji GW, Zhu FP, Xu Q, et al. Radiomic features at contrast-enhanced CT predict recurrence in early stage hepatocellular carcinoma: a multi-institutional study. Radiology 2020;294:568-79.
25. Hectors SJ, Lewis S, Besa C, et al. MRI radiomics features predict immuno-oncological characteristics of hepatocellular carcinoma. Eur Radiol 2020;30:3759-69.
26. Kim J, Choi SJ, Lee SH, Lee HY, Park H. Predicting survival using pretreatment CT for patients with hepatocellular carcinoma treated with transarterial chemoembolization: comparison of models using radiomics. AJR Am J Roentgenol 2018;211:1026-34.
27. Kloth C, Thaiss WM, Kärgel R, et al. Evaluation of texture analysis parameter for response prediction in patients with hepatocellular carcinoma undergoing drug-eluting bead transarterial chemoembolization (DEB-TACE) using biphasic contrast-enhanced ct image data: correlation with liver perfusion CT. Acad Radiol 2017;24:1352-63.
28. Wu LF, Rao SX, Xu PJ, et al. Pre-TACE kurtosis of ADCtotal derived from histogram analysis for diffusion-weighted imaging is the best independent predictor of prognosis in hepatocellular carcinoma. Eur Radiol 2019;29:213-23.
29. Shaghaghi M, Aliyari Ghasabeh M, Ameli S, et al. Post-TACE changes in ADC histogram predict overall and transplant-free survival in patients with well-defined HCC: a retrospective cohort with up to 10 years follow-up. Eur Radiol 2021;31:1378-90.
30. Yang X, Yuan C, Zhang Y, Li K, Wang Z. Predicting hepatocellular carcinoma early recurrence after ablation based on magnetic resonance imaging radiomics nomogram. Medicine 2022;101:e32584.
31. Fiz F, Jayakody Arachchige VS, Gionso M, et al. Radiomics of biliary tumors: a systematic review of current evidence. Diagnostics 2022;12:826.
32. Liu X, Khalvati F, Namdar K, et al. Can machine learning radiomics provide pre-operative differentiation of combined hepatocellular cholangiocarcinoma from hepatocellular carcinoma and cholangiocarcinoma to inform optimal treatment planning? Eur Radiol 2021;31:244-55.
33. Zhou C, Wang Y, Ma L, Qian X, Yang C, Zeng M. Combined hepatocellular carcinoma-cholangiocarcinoma: MRI features correlated with tumor biomarkers and prognosis. Eur Radiol 2022;32:78-88.
34. Ji GW, Zhu FP, Zhang YD, et al. A radiomics approach to predict lymph node metastasis and clinical outcome of intrahepatic cholangiocarcinoma. Eur Radiol 2019;29:3725-35.
35. Chu H, Liu Z, Liang W, et al. Radiomics using CT images for preoperative prediction of futile resection in intrahepatic cholangiocarcinoma. Eur Radiol 2021;31:2368-76.
36. Qin H, Hu X, Zhang J, et al. Machine-learning radiomics to predict early recurrence in perihilar cholangiocarcinoma after curative resection. Liver Int 2021;41:837-50.
37. Groot Koerkamp B, Wiggers JK, Allen PJ, et al. American joint committee on cancer staging for resected perihilar cholangiocarcinoma: a comparison of the 6th and 7th editions. HPB 2014;16:1074-82.
38. Jarnagin WR, Fong Y, DeMatteo RP, et al. Staging, resectability, and outcome in 225 patients with hilar cholangiocarcinoma. Ann Surg 2001;234:507-19.
39. Gazzaniga GM, Faggioni A, Filauro M. Surgical treatment of proximal bile duct tumors. Int Surg 1985;70:45-8.
40. Park HJ, Park B, Park SY, et al. Preoperative prediction of postsurgical outcomes in mass-forming intrahepatic cholangiocarcinoma based on clinical, radiologic, and radiomics features. Eur Radiol 2021;31:8638-48.
41. Zhang J, Wu Z, Zhao J, et al. Intrahepatic cholangiocarcinoma: MRI texture signature as predictive biomarkers of immunophenotyping and survival. Eur Radiol 2021;31:3661-72.
42. Mosconi C, Cucchetti A, Bruno A, et al. Radiomics of cholangiocarcinoma on pretreatment CT can identify patients who would best respond to radioembolisation. Eur Radiol 2020;30:4534-44.
43. Fiz F, Viganò L, Gennaro N, et al. Radiomics of liver metastases: a systematic review. Cancers 2020;12:2881.
44. Wesdorp NJ, van Goor VJ, Kemna R, et al. Advanced image analytics predicting clinical outcomes in patients with colorectal liver metastases: a systematic review of the literature. Surg Oncol 2021;38:101578.
45. Jia LL, Zhao JX, Zhao LP, Tian JH, Huang G. Current status and quality of radiomic studies for predicting KRAS mutations in colorectal cancer patients: a systematic review and meta-analysis. Eur J Radiol 2023;158:110640.
46. Kelahan LC, Kim D, Soliman M, et al. Role of hepatic metastatic lesion size on inter-reader reproducibility of CT-based radiomics features. Eur Radiol 2022;32:4025-33.
47. Ahn SJ, Kim JH, Park SJ, Han JK. Prediction of the therapeutic response after FOLFOX and FOLFIRI treatment for patients with liver metastasis from colorectal cancer using computerized CT texture analysis. Eur J Radiol 2016;85:1867-74.
48. Dercle L, Lu L, Schwartz LH, et al. Radiomics response signature for identification of metastatic colorectal cancer sensitive to therapies targeting EGFR pathway. J Natl Cancer Inst 2020;112:902-12.
49. Dohan A, Gallix B, Guiu B, et al. Early evaluation using a radiomic signature of unresectable hepatic metastases to predict outcome in patients with colorectal cancer treated with FOLFIRI and bevacizumab. Gut 2020;69:531-9.
50. Creasy JM, Midya A, Chakraborty J, et al. Quantitative imaging features of pretreatment CT predict volumetric response to chemotherapy in patients with colorectal liver metastases. Eur Radiol 2019;29:458-67.
51. Abunahel BM, Pontre B, Kumar H, Petrov MS. Pancreas image mining: a systematic review of radiomics. Eur Radiol 2021;31:3447-67.
52. Gao Y, Cheng S, Zhu L, et al. A systematic review of prognosis predictive role of radiomics in pancreatic cancer: heterogeneity markers or statistical tricks? Eur Radiol 2022;32:8443-52.
53. Staal FCR, Aalbersberg EA, van der Velden D, et al. GEP-NET radiomics: a systematic review and radiomics quality score assessment. Eur Radiol 2022;32:7278-94.
54. Goggins M, Overbeek KA, Brand R, et al. Management of patients with increased risk for familial pancreatic cancer: updated recommendations from the International Cancer of the Pancreas Screening (CAPS) Consortium. Gut 2020;69:7-17.
55. Signoretti M, Bruno MJ, Zerboni G, Poley JW, Delle Fave G, Capurso G. Results of surveillance in individuals at high-risk of pancreatic cancer: a systematic review and meta-analysis. United Eur Gastroent J 2018;6:489-99.
56. Paiella S, Capurso G, Cavestro GM, et al. Results of first-round of surveillance in individuals at high-risk of pancreatic cancer from the AISP (Italian Association for the Study of the Pancreas) registry. Am J Gastroenterol 2019;114:665-70.
57. Chhoda A, Vodusek Z, Wattamwar K, et al. Late-stage pancreatic cancer detected during high-risk individual surveillance: a systematic review and meta-analysis. Gastroenterology 2022;162:786-98.
58. Chu LC, Park S, Kawamoto S, et al. Utility of CT radiomics features in differentiation of pancreatic ductal adenocarcinoma from normal pancreatic tissue. AJR Am J Roentgenol 2019;213:349-57.
59. Qureshi TA, Gaddam S, Wachsman AM, et al. Predicting pancreatic ductal adenocarcinoma using artificial intelligence analysis of pre-diagnostic computed tomography images. Cancer Biomark 2022;33:211-7.
60. Javed S, Qureshi TA, Gaddam S, et al. Risk prediction of pancreatic cancer using AI analysis of pancreatic subregions in computed tomography images. Front Oncol 2022;12:1007990.
61. Mukherjee S, Patra A, Khasawneh H, et al. Radiomics-based machine-learning models can detect pancreatic cancer on prediagnostic computed tomography scans at a substantial lead time before clinical diagnosis. Gastroenterology 2022;163:1435-46.e3.
62. Jeon SK, Kim JH, Yoo J, Kim JE, Park SJ, Han JK. Assessment of malignant potential in intraductal papillary mucinous neoplasms of the pancreas using MR findings and texture analysis. Eur Radiol 2021;31:3394-404.
63. Chakraborty J, Midya A, Gazit L, et al. CT radiomics to predict high-risk intraductal papillary mucinous neoplasms of the pancreas. Med Phys 2018;45:5019-29.
64. Permuth JB, Choi J, Balarunathan Y, et al. Combining radiomic features with a miRNA classifier may improve prediction of malignant pathology for pancreatic intraductal papillary mucinous neoplasms. Oncotarget 2016;7:85785-97.
65. Zhang H, Meng Y, Li Q, et al. Two nomograms for differentiating mass-forming chronic pancreatitis from pancreatic ductal adenocarcinoma in patients with chronic pancreatitis. Eur Radiol 2022;32:6336-47.
66. Wei R, Lin K, Yan W, et al. Computer-aided diagnosis of pancreas serous cystic neoplasms: a radiomics method on preoperative MDCT images. Technol Cancer Res Treat 2019;18:1533033818824339.
67. Chang N, Cui L, Luo Y, Chang Z, Yu B, Liu Z. Development and multicenter validation of a CT-based radiomics signature for discriminating histological grades of pancreatic ductal adenocarcinoma. Quant Imaging Med Surg 2020;10:692-702.
68. Tikhonova VS, Karmazanovsky GG, Kondratyev EV, et al. Radiomics model-based algorithm for preoperative prediction of pancreatic ductal adenocarcinoma grade. Eur Radiol 2023;33:1152-61.
69. Gu D, Hu Y, Ding H, et al. CT radiomics may predict the grade of pancreatic neuroendocrine tumors: a multicenter study. Eur Radiol 2019;29:6880-90.
70. De Robertis R, Maris B, Cardobi N, et al. Can histogram analysis of MR images predict aggressiveness in pancreatic neuroendocrine tumors? Eur Radiol 2018;28:2582-91.
71. Mori M, Palumbo D, Muffatti F, et al. Prediction of the characteristics of aggressiveness of pancreatic neuroendocrine neoplasms (PanNENs) based on CT radiomic features. Eur Radiol 2023;33:4412-21.
72. Salinas-Miranda E, Healy GM, Grünwald B, et al. Correlation of transcriptional subtypes with a validated CT radiomics score in resectable pancreatic ductal adenocarcinoma. Eur Radiol 2022;32:6712-22.
73. Bailey P, Chang DK, Nones K, et al. Genomic analyses identify molecular subtypes of pancreatic cancer. Nature 2016;531:47-52.
74. Rigiroli F, Hoye J, Lerebours R, et al. CT radiomic features of superior mesenteric artery involvement in pancreatic ductal adenocarcinoma: a pilot study. Radiology 2021;301:610-22.
75. Bian Y, Guo S, Jiang H, et al. Relationship between radiomics and risk of lymph node metastasis in pancreatic ductal adenocarcinoma. Pancreas 2019;48:1195-203.
76. De Robertis R, Geraci L, Tomaiuolo L, et al. Liver metastases in pancreatic ductal adenocarcinoma: a predictive model based on CT texture analysis. Radiol Med 2022;127:1079-84.
77. Tang TY, Li X, Zhang Q, et al. Development of a novel multiparametric MRI radiomic nomogram for preoperative evaluation of early recurrence in resectable pancreatic cancer. J Magn Reson Imaging 2020;52:231-45.
78. De Robertis R, Tomaiuolo L, Pasquazzo F, et al. Correlation between ADC histogram-derived metrics and the time to metastases in resectable pancreatic adenocarcinoma. Cancers 2022;14:6050.
79. Kulkarni A, Carrion-Martinez I, Jiang NN, et al. Hypovascular pancreas head adenocarcinoma: CT texture analysis for assessment of resection margin status and high-risk features. Eur Radiol 2020;30:2853-60.
80. De Robertis R, Beleù A, Cardobi N, et al. Correlation of MR features and histogram-derived parameters with aggressiveness and outcomes after resection in pancreatic ductal adenocarcinoma. Abdom Radiol 2020;45:3809-18.
81. Kim HS, Kim YJ, Kim KG, Park JS. Preoperative CT texture features predict prognosis after curative resection in pancreatic cancer. Sci Rep 2019;9:17389.
82. Xie T, Wang X, Li M, Tong T, Yu X, Zhou Z. Pancreatic ductal adenocarcinoma: a radiomics nomogram outperforms clinical model and TNM staging for survival estimation after curative resection. Eur Radiol 2020;30:2513-24.
83. Sandrasegaran K, Lin Y, Asare-Sawiri M, Taiyini T, Tann M. CT texture analysis of pancreatic cancer. Eur Radiol 2019;29:1067-73.
84. Healy GM, Salinas-Miranda E, Jain R, et al. Pre-operative radiomics model for prognostication in resectable pancreatic adenocarcinoma with external validation. Eur Radiol 2022;32:2492-505.
85. Borhani AA, Dewan R, Furlan A, et al. Assessment of response to neoadjuvant therapy using CT texture analysis in patients with resectable and borderline resectable pancreatic ductal adenocarcinoma. AJR Am J Roentgenol 2020;214:362-9.
86. Kim BR, Kim JH, Ahn SJ, et al. CT prediction of resectability and prognosis in patients with pancreatic ductal adenocarcinoma after neoadjuvant treatment using image findings and texture analysis. Eur Radiol 2019;29:362-72.
Cite This Article
Export citation file: BibTeX | RIS
OAE Style
De Robertis R, Todesco M, Autelitano D, Spoto F, D’Onofrio M. The role of radiomics in hepato-bilio-pancreatic surgery: a literature review. Art Int Surg 2023;3:166-79. http://dx.doi.org/10.20517/ais.2023.18
AMA Style
De Robertis R, Todesco M, Autelitano D, Spoto F, D’Onofrio M. The role of radiomics in hepato-bilio-pancreatic surgery: a literature review. Artificial Intelligence Surgery. 2023; 3(3): 166-79. http://dx.doi.org/10.20517/ais.2023.18
Chicago/Turabian Style
De Robertis, Riccardo, Marco Todesco, Daniele Autelitano, Flavio Spoto, Mirko D’Onofrio. 2023. "The role of radiomics in hepato-bilio-pancreatic surgery: a literature review" Artificial Intelligence Surgery. 3, no.3: 166-79. http://dx.doi.org/10.20517/ais.2023.18
ACS Style
De Robertis, R.; Todesco M.; Autelitano D.; Spoto F.; D’Onofrio M. The role of radiomics in hepato-bilio-pancreatic surgery: a literature review. Art. Int. Surg. 2023, 3, 166-79. http://dx.doi.org/10.20517/ais.2023.18
About This Article
Special Issue
Copyright
Data & Comments
Data
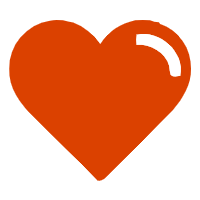

Comments
Comments must be written in English. Spam, offensive content, impersonation, and private information will not be permitted. If any comment is reported and identified as inappropriate content by OAE staff, the comment will be removed without notice. If you have any queries or need any help, please contact us at support@oaepublish.com.