The current use of artificial intelligence in testicular cancer: a systematic review
Abstract
Testicular cancer is often overshadowed by other cancers despite being the most common cancer in men aged 15 to 34 years. This systematic review focuses on the potential of machine learning and deep learning techniques in the areas of testicular cancer imaging and histopathology, where artificial intelligence (AI) could assist in diagnosis, evaluation, and prognostication. Various studies have highlighted AI’s ability to accurately distinguish between benign and malignant lesions and characterisation within malignant lesions using magnetic resonance imaging (MRI) radiomics. Models have also been used in predicting histopathological findings to allow for greater accuracy and reproducibility. Further work is required to explore AI implementation in ultrasound imaging, which is the cheapest and most used modality.
Keywords
INTRODUCTION
The term “artificial intelligence” (AI) was first coined for the Dartmouth Summer Workshop in 1956, where it was broadly referred to as “thinking machines”[1]. Artificial intelligence can be categorised into machine learning (ML) and deep learning (DL). Machine learning utilises statistical models to generate algorithms to assess data and variables, referred to as “features”, to predict outcomes. With further data input, machine learning can improve its own performance over time.
The application of AI is vast and expanding rapidly in oncology[2] with the use of machine learning and deep learning. Interestingly, research as early as 1995 used deep learning neural networks to quantitate primary tumour to determine if more accurate staging could be achieved, which showed promising results[3]. Since then, early adoption of AI in urology has been beneficial, with a particular focus on renal, bladder, and prostate cancer[4,5]. However, testicular cancer is often overshadowed by the three aforementioned cancers, despite being the most common cancer in men aged 15 to 34 years[6], with annual incidence estimated to reach 23,000 in Europe by 2025, an increase of 24% from 2004[7,8].
Testicular cancer
The WHO classification of testicular cancer broadly distinguishes between germ cell neoplasia in situ (GCNIS; 95%) and non-GCNIS (5%), with the former further subdivided into seminomas and
In conjunction with imaging, three important blood serum tumour markers carry diagnostic value at initial diagnosis and after orchidectomy - serum alpha-fetoprotein (AFP), beta subunit of human chorionic gonadotropin (beta-HCG), and lactate dehydrogenase (LDH). Elevation of these three tumour markers has been seen in up to 60% of patients[7]. Post-orchidectomy tumours markers, staging CT scan results, and histopathological results are used to classify patients in TMN (tumour, metastasis, and lymph node involvement) classification and their prognosis. Patients with lymphovascular invasion, typically involving retroperitoneal lymph nodes, have a higher risk of recurrence of cancer[16]. These are central to the use of AI in testicular cancer.
Machine learning and deep learning
Machine learning predominantly uses either supervised or unsupervised learning. Supervised learning is frequently employed by research teams when there is a clearly defined outcome, often of a binary nature, such as distinguishing between malignant and benign cases. The data requires meticulous annotation, a labour-intensive pre-processing endeavour, which prepares the dataset for the algorithm. Commonly used supervised learning ML algorithms include, but are not confined to, linear regression, random forest, and support vector machines (SVM). The concept of train-test split is fundamental to supervised machine learning; 70%-80% of the dataset is allocated to a training set and the remaining 20%-30% is used to validate the performance of the training model. In addition, cross-validation can be used and is most useful in smaller datasets and mitigates overfitting (an issue whereby machine learning does well in the training cohort but less well in unseen data in the testing cohort)[17,18].
On the other hand, unsupervised learning does not involve labelled output data and is used to identify commonalities of “features” within the dataset. In clinical practice, this type of learning is useful in identifying unrecognised patterns, clusters, or feature subsets, and commonly used algorithms include
METHODS
This review was designed and performed in accordance with the recommendations of the Preferred Reporting Items for Systematic reviews and Meta-Analyses (PRISMA) guidelines.
Inclusion criteria
- Original research full articles relevant to artificial intelligence including machine learning and deep learning in testicular cancer, seminomas, or germ cell tumours.
- Live human study participants
Exclusion criteria
- Grey literature
- Abstracts from conferences
- Non-peer-reviewed articles
- Non-English articles
- Animal studies
- Paediatric studies
Literature search and study selection
A systematic search of Cochrane CENTRAL database, MEDLINE, EMBASE, NHS NICE healthcare databases advanced search interface was executed by two authors (Chuluunbaatar Y and Bansal S). The last search was conducted on the 28th of June 2023. Medical subject headings (MeSH) terms were used - “artificial intelligence testicular cancer”, “machine learning testicular cancer”, and “artificial learning seminoma germ cell tumour”. Two independent screeners performed a title and abstract review, with a third screener to resolve any disagreements (Brodie A).
Data collection
For each study included in this systematic review, the following data was extracted: the application, imaging technique where applicable, cancer type, sample size, training cohort size, validation cohort size, machine learning or deep learning AI algorithm, accuracy, sensitivity, specificity, and/or area under curve (AUC). Data extraction was performed independently by two authors (Chuluunbaatar Y, Bansal S), and disagreements were resolved by discussion and consensus. If no agreement could be reached, a third author (Brodie A) was consulted to act as an arbitrator. Studies with missing data were excluded from the systematic review.
Assessment for risk of bias
Two authors (Chuluunbaatar Y, Bansal S) critically appraised the methodological quality of the included studies. Discrepancies were resolved by reviewer discussion, and if they remained unsolved, a third investigator was consulted (Brodie A).
Search result: 102 citations were identified. Following screening and assessment, 9 studies were included in the review [Figure 1].
RESULTS
AI in testicular cancer imaging
Different imaging modalities are important in the diagnostic work-up and initial staging of testicular cancer. While ultrasound is essential in diagnosis, it cannot differentiate benign from malignant types[20], and thus, limited work on AI and ultrasound has been carried out.
Recent studies involving MRI have been promising. Fan et al. developed a supervised machine learning-based approach to distinguish malignant and benign testicular masses using diffusion weight imaging (DWI) MRI “radiomic” signatures based on appearance diffusion coefficient (ADC) maps[21]. Radiomics refers to a widely used method of extracting quantitative features or data from digital medical images into high-dimensional mineable data[22]. In 97 patients with 101 testicular lesions, 851 distinct radiomic features were extracted from ADC maps. A predictive model was trained using a subset (71 lesions) and included 272 features, of which six were statistically significant and input into the AI algorithm. These performed well in a separate validation cohort [30 lesions and area under the curve (AUC) = 0.868] and, therefore, were able to distinguish malignant vs. benign masses well. The study also analysed blood tests, which showed that levels of serum markers (AFP, hCG, and LDH) were increased in 67% of testicular malignancies in their training and validation cohorts. Further statistical analysis showed that it was not an independent factor for testicular malignancy.
Furthermore, Zhang et al. used minimum-redundancy maximum-relevance (mRMR) together with least absolute shrinkage and selection operator (LASSO), supervised machine learning algorithms, to design a classifier for seminomas and non-seminomas in 39 patients with germ-cell tumours using their T2-weighted MRI images prior to radical orchiectomy[15]. As a labelled dataset, 19 patients were known to have seminomas and the remainder with non-seminomas. From these MRI images of 39 patients, a total of 851 radiomic features were extracted, of which features were ranked using the mRMR method according to their relevance-redundancy scores. Following this and 5-fold cross-validation, five features were included in the radiomic signature. The algorithms were able to statistically distinguish between seminomas and
Similarly, Feliciani et al. investigated the role of radiomics in 42 patients with histology-proven testicular neoplasms to differentiate between germ-cell tumours and non-germ cell tumours and between seminomas and non-seminomas within germ-cell tumours using T2-weighted MRI images prior to surgery[20]. From 42 patients, 44 lesions were identified, and 487 features extracted. LASSO algorithm was applied and identified three radiomic features for the discrimination of germ-cell vs. non-germ cell tumours and four radiomic features for discrimination of seminoma vs. non-seminoma. These radiomic features were applied to a linear support vector machine (SVM) algorithm, a supervised machine learning algorithm. They showed that the model produced a true positive rate (TPR) of 94% and 74% in predicting germ-cell tumours and non-germ cell tumours, respectively. The F-score for germ-cell tumours is 0.92 and 0.78 for non-germ cell tumours, which is a score that provides a balanced measure of the model’s precision and recall. Within germ-cell tumours, the model was able to predict seminomas and non-seminomas with a TPR of 86% and 87%, respectively.
In addition to MRI, CT imaging has also been implemented in AI algorithms. Baessler et al. explored the potential for CT imaging to distinguish between benign and malignant lymph node involvement in 80 patients with metastatic non-seminomas who underwent post-chemotherapy retroperitoneal lymph node dissection (PC-RPLND)[23]. They used gradient boost tree (GBT), a supervised machine learning algorithm, to develop a radiomics-based predictive model that achieved a classification accuracy of 0.81 compared to histological assessment. This study further solidified research carried out by Lewin et al. in which they used CT radiomics from 77 patients with PC-RPLND to distinguish residual masses as either malignant or benign (fibrotic) using supervised machine learning SVM[24]. Ten radiomics features were identified and the algorithm performed well with an AUC of 0.80 compared with pathological reports.
These studies highlight the potential role AI can play in assisting diagnosis and classification via imaging. Further investigation is required to explore AI implementation in ultrasound imaging, the cheapest and commonly used modality.
AI in testicular cancer histopathology
Histological assessment by pathologists is prone to inter- and intra-observer bias. AI within histopathology has advanced exponentially and allows for greater accuracy and more reproducible results[25,26].
Tumour-infiltrating lymphocytes (TILs) have prognostic significance in testicular germ cell tumours such that their presence often indicates poorer prognosis[27,28], which are typically quantified histologically following haematoxylin and eosin (H&E) staining. Linder et al. utilised deep learning algorithms (commercially available but not specified) to assist in the detection of TILs in testicular germ cells in 259 specimens obtained retrospectively from 118 patients[29]. The algorithm initially trained with 129 specimens, with the remaining 130 specimens reserved for testing, where the model performed with a sensitivity of 89% and F-score of 0.88 compared to manually annotated TILs. This showed high detection levels with good accuracy and precision.
In a similar fashion, Ghosh et al. carried out research on lymphovascular invasion (LVI) in 29 germ-cell testicular cancer patients retrospectively by using 184 whole slide images from primary management with orchidectomy retrospectively[30]. The expertly annotated images were input into “Visiopham” AI module, which utilises deep learning convolutional neural network (CNN) for training. The model was applied to a test data set of 43 slides. The model demonstrated that it was able to identify lymphovascular invasion with an accuracy of 0.56. However, a few foci that the AI model identified as non-LVI were re-reviewed by an expert and determined to be in fact LVI. Although these models are not perfectly precise, they set the foundation for future work and highlight the importance of larger datasets required for stronger training and validation of AI models. It is particularly important as LVI is considered an important prognostic marker in non-seminomas, with a relapse rate of 50% if present[31].
AI in testicular cancer outcomes
A plethora of machine learning techniques have been devised to prognosticate patient outcomes, such as “DeepSurv”[32], “DeepHit”[33], and recently “Deep Survival Machine” models[34]. However, these methods of estimating the survival distribution fail to provide insight into post-prognosis surveillance. To bridge this gap, Eminaga et al. used data from “Surveillance, Epidemiology and End Results (SEER) program” encompassing a vast cohort of cancer patients (penile, testicular, prostate, bladder, ureter, and kidney) to construct a machine learning model capable of dynamic risk-stratification for patient survival and
A small proportion of patients develop advanced disease stages characterised by metastases, typically to the lung followed by the liver[36]. In a concerted effort to unravel the underlying risk factors and predict which patients are more susceptible to metastasis, machine learning algorithms are being harnessed. Recently, a study leveraged five supervised machine learning algorithms (linear regression, extreme gradient boosting, light gradient boosting, random forest, and k-nearest neighbours) and one deep learning algorithm (multilayer protection) to accurately prognosticate metastatic disease (M1B progression) in patients with GCTC. The study employed 4,300 patients from the SEER program database and evaluated various potential factors through statistical analysis[37]. The results of the study revealed that T-stage, N-stage, lung metastases and distant lymph node metastases exerted the most statistically significant impact on M1B progression and therefore incorporated into the machine learning algorithm. XGBoost was identified as the most effective algorithm for forecasting metastatic risk, demonstrating an AUC of 0.957. Serum markers (AFP, LDH, and hCG) were also identified as not being a predictor of progression to metastatic stage. Despite the results, it is essential to note that immunohistochemistry findings were not factored into the algorithm, thereby leaving room for further valuable input into identifying risk factors.
DISCUSSION
This review has summarised recent novel research in the utilisation of AI in testicular cancer, as summarised in Table 1. As testicular cancer accounts for approximately 1% of all new cancer diagnoses in the world[6], it is an uncommon cancer, and therefore, this is reflected in the small sample sizes of the vast majority of studies carried out. Consequently, many studies conducted feature small sample sizes. As a corollary, several studies lack a validation or testing cohort to test their algorithm [Table 1], and thus rely heavily on cross-validation. Multiple authors have acknowledged this as a common limitation, emphasizing the need for larger datasets to generate accurate predictions. This is particularly crucial in non-seminoma tumours, which are characterised by greater heterogeneity than seminomas, necessitating a greater number of samples to enable deep learning to assimilate a broader range of patterns.
Summary of the studies involved in AI and testicular cancer
Study | Application | Study summary | Study type & dates | Sample | Limitations | Algorithm/Model | Accuracy % | Sensi-tivity % | Speci-ficity % | AUC |
Fan et al., 2022[21] | Detection | Develop machine learning-based radiomics to discriminate between benign and malignant testicular masses DWI MRI images | Retrospective single-site study from February 2014 to April 2021 | 97 patients with 101 testicular masses Extracted = 851 radiomics 272 statistically different 6 features used Training cohort = 71 Validation cohort = 30 | Small training and validation cohorts | Supervised machine learning Did not explicitly mention | NR | 0.905 | 0.667 | 0.868 |
Zhang | Imaging | T2-weighted image-based radiomics for differentiating between seminoma and non-seminoma | Retrospective single-site study from February 2014 to March 2019 | 39 patients Pathologically identified lesions Five features selected as radiomic signatures | There is no training or validation cohort due to the small sample size. Instead, they used cross-validation | Supervised machine learning mRMR LASSO | NR | 90 | 100 | 0.979 |
Feliciani | Imaging | Using MRI (T2-weighted) based radiomics to distinguish between germ-cell tumours vs. non-germ cell tumours To distinguish between seminoma and non-seminoma cancers Against known histological findings | Retrospective single-site study between January 2006 and February 2019 | 42 patients 44 lesions 487 features extracted 3 radiomic features used in germ-cell vs. non-germ cell tumours 4 radiomic features used in seminoma vs. non-seminoma | No training or validation cohort due to the small sample size. Cross-validation used to mitigate this Retrospective | Supervised machine learning Linear model SVM | 89% | NR | NR | NR |
Baessler et al., 2020[23] | Imaging | CT radiomics-based machine learning to predict histopathology of LN after dissection in metastatic non-seminoma Compared against histopathological findings | Retrospective single-site study between 2008 and 2017 | 80 patients with 204 lymph nodes 97 radiomics features per lymph node. 5 features used in AI algorithm Training dataset = 120 LNs Testing dataset = 23 LNs Validation dataset = 61 LNs | Various CT scanners used Did not include clinical variables in the AI algorithm | Supervised machine learning GBT | 0.81 | 88 | 72 | NR |
Lewin | Imaging | Contrast CT radiomics in predicting post-chemotherapy residual mass and compared with pathological reports | Retrospective single-site study between January | 77 patients 183 radiomics 10 statistical | No validation or training datasets | Supervised machine learning Support vector machine | 72 | 56 | 82 | 0.80 |
Ghosh | Histopathology | Detection of lymphovascular invasion in testicular cancer | Retrospective single-site study from January 2019 to July 2020 | 29 patients 302 whole haematoxylin and eosin slides Training set = 184 whole slides from 19 patients. Validation set = 118 whole slides from 10 patients | Small number of cases | Deep learning Convolutional neural network | 0.68 | NR | NR | NR |
Linder | Histopathology | Detecting tumour-infiltrating lymphocytes from H&E slides | Retrospective single-site study between 2005 and 2016 | 89 patients 28 whole slide H&E tissue samples 259 images created Training = 129 images Testing = 130 images | Small sample size | Deep learning Commercially available image analysis software WebMicroscope | NR | 89 | NR | |
Eminaga et al., 2022[35] | Outcomes | Prognostic and follow-up recommendations | Retrospective study utilising a US SEER National database with 1.9 million patients with urological cancers between 1975 and 2017 | 1.9 million patients Training set = 1.7 million patients Test set = 194,000 patients | SEER database does not include detailed treatment information and has evolving standard-of-care treatment | Deep learning Recurrent neural network | 0.8 | NR | NR | NR |
Ding et al., 2022[37] | Outcomes | Predicting the risk of developing metastatic disease with germ-cell testicular cancer | Retrospective study US SEER national database | SEER database Total 4,323 patients | Lack of immuno-histochemistry results and patients with underlying disease and | Supervised machine learning Multiple | NR | NR | NR | 0.816 with random forest |
The use of CT and MRI imaging radiomics is an emerging field in testicular cancer. One study used diffusion-weighted imaging MRI modality, while others used the commonly used T2-weighted MRI modality. Diffusion-weighted images are quantitatively assessed by calculating the ADC maps and scores, which offer information regarding cellular structure and organisation of tissues and, therefore, delineate between conditions, such that tissues with higher cell density, i.e., cancers, tend to have lower ADC values and vice versa[38]. DWI, however, suffers from certain limitations such as geometric distortion and signal intensity dropout on tissue-air boundaries, such as thyroid gland, scrotum and prostate[15]. Nevertheless, studies have demonstrated that DWI alone confers powerful diagnostic performance in testicular masse, with one study reporting sensitivity of 90% and specificity of 88%[39]. Moreover, the amalgamation of ADC and conventional T2-weighed MRI has further improved diagnostics[40,41]. The combination of the two has yet to be explored in AI algorithms and thereby an open scope for further investigation in this direction.
Despite a focus on CT and MRI radiomics, no current literature exists pertaining to the role of AI in ultrasound in testicular cancer, which is the first-line imaging modality. The use of AI in ultrasound has been predominantly explored in thyroid and breast, followed by cardiac, vascular, obstetrics and gynaecology, and musculoskeletal system[42]. For instance, in the context of thyroid nodules, AI has been used to aid in the diagnosis of malignant and benign nodules, classify malignant modules, and prognosticate outcomes[43]. In a study by Liu et al., a novel deep-learning CNN computer-aided model was employed to detect and classify thyroid nodules in ultrasound images[44]. The deep learning model surpassed the diagnostic capabilities of radiologists as observed in sensitivity (0.964 vs. 0.928), specificity (0.780 vs. 0.366), and accuracy (0.928 vs. 0.816). Given both thyroid and testicular masses are investigated first line using ultrasound, these studies demonstrate the possible applications of US in testicular cancer. As such, this constitutes a critical area of investigation for future research.
Additionally, serum markers play a pivotal role in the diagnosis and prognostication of testicular, albeit as a non-independent marker[7,37]. The emerging field of serum biomarkers and proteomics with machine learning is an exciting advancement and has been demonstrated in the prediction and prognosis of hepatocellular carcinoma (HCC)[45]. Lee et al. demonstrated the use of machine learning with cell-free DNA (cfDNA), specifically AFP expression, detection system to establish a platform for HCC diagnosis and prognosis[46]. Their approach showed improved accuracy in determining pathological features of HCC using a composite cfDNA score (which integrated total plasma cfDNA and cfAFP-DNA expression into a single score). Similar strategies could potentially be employed in the realm of testicular cancer with AFP, LDH, and hCG markers. Further research is warranted to explore the possibility of this.
There are several limitations of the papers and data included worth noting. Primarily, these limitations stem from the novelty of using AI algorithms within research such that the studies incorporated in this systematic review carried out their work retrospectively. This subsequently means that primarily internal validation processes are employed. However, for a more comprehensive assessment of these AI algorithms, external validation is imperative following testing with larger datasets to enable a better understanding of their performance in real-world scenarios with unseen data in prospective patients. Additionally, the retrospective nature of the studies often necessitated the resampling of images in order to address the heterogeneity of images to create a more uniform dataset. However, it is worth noting that this process may not perfectly mirror the diverse range of imaging equipment used across different clinical centres.
In essence, while these limitations are inherent to the current state of AI research and its vastly developing field, they provide invaluable insights into the challenges and opportunities for further development and validation of promising AI technologies within a clinical context.
CONCLUSION
The diverse array of studies employing AI techniques to address various aspects of testicular cancer underscores the extensive scope and versatility of AI in facilitating diagnosis, classification, and prognostication, ultimately impacting patient care. However, it is worth noting that a significant challenge associated with machine learning is its dependence on large-scale training and testing datasets to ensure the creation of robust and generalisable models.
DECLARATIONS
AcknowledgementsAcknowledgement to Roy Zhang for reading the initial manuscript.
Authors’ contributionsMade substantial contributions to the conception and design of the study and performed data analysis and interpretation: Chuluunbaatar Y
Performed data acquisition, as well as providing administrative, technical, and material support: Bansal S, Brodie A, Sharma A, Vasdev N
Availability of data and materialsNot applicable.
Financial support and sponsorshipNone.
Conflicts of interestAll authors declared that there are no conflicts of interest.
Ethical approval and consent to participate.Not applicable.
Consent for publicationNot applicable.
Copyright© The Author(s) 2023.
REFERENCES
1. Russell S, Norvig P. Artificial intelligence: a modern approach. Third edition. Available from: https://people.engr.tamu.edu/guni/csce421/files/AI_Russell_Norvig.pdf. [Last accessed on 9 Oct 2023].
2. Bhinder B, Gilvary C, Madhukar NS, Elemento O. Artificial intelligence in cancer research and precision medicine. Cancer Discov 2021;11:900-15.
3. Moul JW, Snow PB, Fernandez EB, Maher PD, Sesterhenn IA. Neural network analysis of quantitative histological factors to predict pathological stage in clinical stage I nonseminomatous testicular cancer. J Urol 1995;153:1674-7.
4. Brodie A, Dai N, Teoh JY, Decaestecker K, Dasgupta P, Vasdev N. Artificial intelligence in urological oncology: an update and future applications. Urol Oncol 2021;39:379-99.
5. Checcucci E, Autorino R, Cacciamani GE, et al. Artificial intelligence and neural networks in urology: current clinical applications. Minerva Urol Nefrol 2020;72:49-57.
7. Laguna MP, Pizzocaro G, Klepp O, et al. EAU guidelines on testicular cancer. Eur Urol 2001;40:102-10.
8. Le Cornet C, Lortet-Tieulent J, Forman D, et al. Testicular cancer incidence to rise by 25% by 2025 in Europe? Model-based predictions in 40 countries using population-based registry data. Eur J Cancer 2014;50:831-9.
9. Isidori AM, Pozza C, Gianfrilli D, et al. Differential diagnosis of nonpalpable testicular lesions: qualitative and quantitative contrast-enhanced US of benign and malignant testicular tumors. Radiology 2014;273:606-18.
10. Pedersen MR, Sloth Osther PJ, Nissen HD, Vedsted P, Møller H, Rafaelsen SR. Elastography and diffusion-weighted MRI in patients with testicular microlithiasis, normal testicular tissue, and testicular cancer: an observational study. Acta Radiol 2019;60:535-41.
11. Rocher L, Criton A, Gennisson JL, et al. Characterization of testicular masses in adults: performance of combined quantitative shear wave elastography and conventional ultrasound. Ultrasound Med Biol 2019;45:720-31.
12. Pierre T, Selhane F, Zareski E, et al. The Role of CT in the staging and follow-up of testicular tumors: baseline, recurrence and pitfalls. Cancers 2022;14:3965.
13. Parenti GC, Feletti F, Carnevale A, Uccelli L, Giganti M. Imaging of the scrotum: beyond sonography. Insights Imaging 2018;9:137-48.
14. Tsili AC, Sofikitis N, Stiliara E, Argyropoulou MI. MRI of testicular malignancies. Abdom Radiol 2019;44:1070-82.
15. Zhang P, Feng Z, Cai W, et al. T2-weighted image-based radiomics signature for discriminating between seminomas and nonseminoma. Front Oncol 2019;9:1330.
16. Patrikidou A, Cazzaniga W, Berney D, et al. European association of urology guidelines on testicular cancer: 2023 update. Eur Urol 2023;84:289-301.
17. Lammers DT, Eckert CM, Ahmad MA, Bingham JR, Eckert MJ. A surgeon’s guide to machine learning. Ann Surg Open 2021;2:e091.
18. Albahra S, Gorbett T, Robertson S, et al. Artificial intelligence and machine learning overview in pathology & laboratory medicine: a general review of data preprocessing and basic supervised concepts. Semin Diagn Pathol 2023;40:71-87.
19. Morris MX, Rajesh A, Asaad M, Hassan A, Saadoun R, Butler CE. Deep learning applications in surgery: current uses and future directions. Am Surg 2023;89:36-42.
20. Feliciani G, Mellini L, Carnevale A, et al. The potential role of MR based radiomic biomarkers in the characterization of focal testicular lesions. Sci Rep 2021;11:3456.
21. Fan C, Sun K, Min X, et al. Discriminating malignant from benign testicular masses using machine-learning based radiomics signature of appearance diffusion coefficient maps: comparing with conventional mean and minimum ADC values. Eur J Radiol 2022;148:110158.
22. Society of Radiology (ESR). What the radiologist should know about artificial intelligence - an ESR white paper. Insights Imaging 2019;10:44.
23. Baessler B, Nestler T, Pinto Dos Santos D, et al. Radiomics allows for detection of benign and malignant histopathology in patients with metastatic testicular germ cell tumors prior to post-chemotherapy retroperitoneal lymph node dissection. Eur Radiol 2020;30:2334-45.
24. Lewin J, Dufort P, Halankar J, et al. Applying radiomics to predict pathology of postchemotherapy retroperitoneal nodal masses in germ cell tumors. JCO Clin Cancer Inform 2018;2:1-12.
25. Ghoshal B, Hikmet F, Pineau C, Tucker A, Lindskog C. DeepHistoClass: a novel strategy for confident classification of immunohistochemistry images using deep learning. Mol Cell Proteomics 2021;20:100140.
26. Serag A, Ion-Margineanu A, Qureshi H, et al. Translational AI and deep learning in diagnostic pathology. Front Med 2019;6:185.
27. Berney DM, Cree I, Rao V, et al. An introduction to the WHO 5th edition 2022 classification of testicular tumours. Histopathology 2022;81:459-66.
28. Wilkinson PM, Read G. International Germ Cell Consensus Classification: a prognostic factor-based staging system for metastatic germ cell cancers. International Germ Cell Cancer Collaborative Group. J Clin Oncol 1997;15:594-603.
29. Linder N, Taylor JC, Colling R, et al. Deep learning for detecting tumour-infiltrating lymphocytes in testicular germ cell tumours. J Clin Pathol 2019;72:157-64.
30. Ghosh A, Sirinukunwattana K, Khalid Alham N, et al. The potential of artificial intelligence to detect lymphovascular invasion in testicular cancer. Cancers 2021;13:1325.
31. Albers P, Siener R, Kliesch S, et al. Risk factors for relapse in clinical stage I nonseminomatous testicular germ cell tumors: results of the german testicular cancer study group trial. J Clin Oncol 2003;21:1505-12.
32. Katzman JL, Shaham U, Cloninger A, Bates J, Jiang T, Kluger Y. DeepSurv: personalized treatment recommender system using a Cox proportional hazards deep neural network. BMC Med Res Methodol 2018;18:24.
33. Lee C, Zame W, Yoon J, Van der Schaar M. DeepHit: a deep learning approach to survival analysis with competing risks. In: Proceedings of the AAAI Conference on Artificial Intelligence. 2018.
34. Nagpal C, Li X, Dubrawski A. Deep survival machines : fully parametric survival regression and representation learning for censored data with competing risks. IEEE J Biomed Health Inform 2021;25:3163-75.
35. Eminaga O, Shkolyar E, Breil B, et al. Artificial intelligence-based prognostic model for urologic cancers: a SEER-based study. Cancers 2022;14:3135.
36. Xu P, Wang J, Abudurexiti M, et al. Prognosis of patients with testicular carcinoma is dependent on metastatic site. Front Oncol 2019;9:1495.
37. Ding L, Wang K, Zhang C, et al. A machine learning algorithm for predicting the risk of developing to m1b stage of patients with germ cell testicular cancer. Front Public Health 2022;10:916513.
38. Tsili AC, Sofikitis N, Pappa O, Bougia CK, Argyropoulou MI. An overview of the role of multiparametric MRI in the investigation of testicular tumors. Cancers 2022;14:3912.
39. Tsili AC, Argyropoulou MI, Giannakis D, Tsampalas S, Sofikitis N, Tsampoulas K. Diffusion-weighted MR imaging of normal and abnormal scrotum: preliminary results. Asian J Androl 2012;14:649-54.
40. Wang W, Sun Z, Chen Y, et al. Testicular tumors: discriminative value of conventional MRI and diffusion weighted imaging. Medicine 2021;100:e27799.
41. Liu R, Li J, Jiang Y, et al. The utility of diffusion-weighted imaging and ADC values in the characterization of mumps orchitis and seminoma. Acta Radiol 2022;63:416-23.
42. Shen YT, Chen L, Yue WW, Xu HX. Artificial intelligence in ultrasound. Eur J Radiol 2021;139:109717.
43. Bini F, Pica A, Azzimonti L, et al. Artificial intelligence in thyroid field - a comprehensive review. Cancers 2021;13:4740.
44. Liu T, Guo Q, Lian C, et al. Automated detection and classification of thyroid nodules in ultrasound images using clinical-knowledge-guided convolutional neural networks. Med Image Anal 2019;58:101555.
45. Moldogazieva NT, Mokhosoev IM, Zavadskiy SP, Terentiev AA. Proteomic profiling and artificial intelligence for hepatocellular carcinoma translational medicine. Biomedicines 2021;9:159.
Cite This Article
Export citation file: BibTeX | RIS
OAE Style
Chuluunbaatar Y, Bansal S, Brodie A, Sharma A, Vasdev N. The current use of artificial intelligence in testicular cancer: a systematic review. Art Int Surg 2023;3:195-206. http://dx.doi.org/10.20517/ais.2023.26
AMA Style
Chuluunbaatar Y, Bansal S, Brodie A, Sharma A, Vasdev N. The current use of artificial intelligence in testicular cancer: a systematic review. Artificial Intelligence Surgery. 2023; 3(4): 195-206. http://dx.doi.org/10.20517/ais.2023.26
Chicago/Turabian Style
Chuluunbaatar, Yanjinlkham, Saakshi Bansal, Andrew Brodie, Anand Sharma, Nikhil Vasdev. 2023. "The current use of artificial intelligence in testicular cancer: a systematic review" Artificial Intelligence Surgery. 3, no.4: 195-206. http://dx.doi.org/10.20517/ais.2023.26
ACS Style
Chuluunbaatar, Y.; Bansal S.; Brodie A.; Sharma A.; Vasdev N. The current use of artificial intelligence in testicular cancer: a systematic review. Art. Int. Surg. 2023, 3, 195-206. http://dx.doi.org/10.20517/ais.2023.26
About This Article
Special Issue
Copyright
Data & Comments
Data
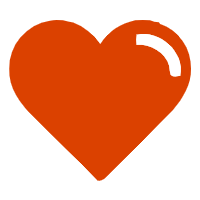

Comments
Comments must be written in English. Spam, offensive content, impersonation, and private information will not be permitted. If any comment is reported and identified as inappropriate content by OAE staff, the comment will be removed without notice. If you have any queries or need any help, please contact us at support@oaepublish.com.