Current state of radiomics in hepatobiliary and pancreatic malignancies
Abstract
Rising in incidence, hepatobiliary and pancreatic (HPB) cancers continue to exhibit dismal long-term survival. The overall poor prognosis of HPB cancers is reflective of the advanced stage at which most patients are diagnosed. Late diagnosis is driven by the often-asymptomatic nature of these diseases, as well as a dearth of screening modalities. Additionally, standard imaging modalities fall short of providing accurate and detailed information regarding specific tumor characteristics, which can better inform surgical planning and sequencing of systemic therapy. Therefore, precise therapeutic planning must be delayed until histopathological examination is performed at the time of resection. Given the current shortcomings in the management of HPB cancers, investigations of numerous noninvasive biomarkers, including circulating tumor cells and DNA, proteomics, immunolomics, and radiomics, are underway. Radiomics encompasses the extraction and analysis of quantitative imaging features. Along with summarizing the general framework of radiomics, this review synthesizes the state of radiomics in HPB cancers, outlining its role in various aspects of management, present limitations, and future applications for clinical integration. Current literature underscores the utility of radiomics in early detection, tumor characterization, therapeutic selection, and prognostication for HPB cancers. Seeing as single-center, small studies constitute the majority of radiomics literature, there is considerable heterogeneity with respect to steps of the radiomics workflow such as segmentation, or delineation of the region of interest on a scan. Nonetheless, the introduction of the radiomics quality score (RQS) demonstrates a step towards greater standardization and reproducibility in the young field of radiomics. Altogether, in the setting of continually improving artificial intelligence algorithms, radiomics represents a promising biomarker avenue for promoting enhanced and tailored management of HPB cancers, with the potential to improve long-term outcomes for patients.
Keywords
INTRODUCTION
Hepatobiliary and pancreatic malignancies comprise a heterogeneous group of diseases that rank amongst the leading causes of cancer-related deaths worldwide[1]. Despite improvements in cancer surveillance, imaging, and treatment, the prognosis of these patients remains poor, with the 5-year survival rate of pancreatic cancer [pancreatic ductal adenocarcinoma (PDAC)] and biliary tract carcinomas (BTCs) reported of only 10% and 5%-18%, respectively[2,3]. The prognosis of localized hepatocellular carcinoma (HCC) is marginally better at 33%; however, it drops off to 2% in the context of metastatic disease[4].
Poor outcomes are predominantly driven by a delay in the diagnosis of these diseases[1]. Diagnostic challenges of hepatobiliary and pancreatic (HPB) malignancies are multifaceted. Firstly, due to the asymptomatic nature of the disease, these patients are diagnosed with more advanced diseases, with only 43% of hepatic cancer and 20% of pancreatic cancers being diagnosed at an early stage[5,6]. Secondly, there is a lack of screening modalities (PDAC) or the current screening strategies are not very accurate (HCC), e.g., the ultrasound-based protocol for HCC has a sensitivity of 47%-63% for detection of early-stage disease[7]. Biomarkers for screening of these diseases are lacking[8,9]. Additionally, pancreatic cancer often mimics benign lesions on imaging, which can result in misdiagnosis and delay in the delivery of appropriate care[6]. It is estimated that nearly 5%-11% of all patients undergoing pancreaticoduodenectomies for pancreatic cancer turn out to have benign lesions[10]. Altogether, there is a critical need for noninvasive biomarkers to facilitate earlier diagnosis of hepatobiliary and pancreatic malignancies.
Once a diagnosis is established, tumor characterization (tumor grade, presence of nodal disease, extent of local invasion, and molecular profile) is essential in determining appropriate care. This is vital for sequencing of systemic therapy and surgical planning[11,12]. While helpful, current imaging modalities are limited by their accuracy in determining these features. Furthermore, the selection of appropriate systemic therapies presents a challenge in the management of these diseases. Currently, clinical tools to predict treatment response are absent, and it is not determined until the patient undergoes resection and a histopathological examination of the specimen is performed. If the disease was resistant to the administered chemotherapeutics, unfortunately, the patient gained no benefit from this therapy, while allowing time for resistant clones to proliferate and result in the progression of disease. For instance, in the setting of metastatic PDAC, Nab-paclitaxel combined with gemcitabine represents the standard of care; however, there is considerable heterogeneity among patients with respect to duration of treatment (0.1-21.9 months), secondary to the significant toxicities of these therapies, as demonstrated by the MPACT trial, as well as treatment response, with the SIEGE trial reporting almost a fifth of patients failing to reach their first treatment response assessment[13,14]. As such, there is a critical need to robustly validate biomarkers to both spare patients from toxicities associated with treatments that they are unlikely to respond to and to identify new avenues for targeted treatments.
Recently, multiple biomarkers have shown promise in hepatobiliary and pancreatic malignancies, including circulating tumor cells, circulating tumor DNA, proteins, and radiomics[9,15-17]. The identification and validation of biomarkers could aid in patient stratification, treatment planning, and prediction of response to therapy and risk of recurrence, thereby improving survival[18]. This narrative review focuses on the current literature on radiomics as a biomarker for hepatobiliary and pancreatic malignancies. We discuss how radiomics could help guide the management of these diseases, the current limitations of radiomics, and future applications and integration in the clinical setting.
METHODS
To identify and synthesize literature for a narrative review regarding the utility and applications of radiomics in the management of HPB tumors, PubMed and Embase, as well as Google Scholar, were queried. These platforms were searched from inception until July 2023. Given that a qualitative review was planned, all article types published in English were considered eligible for inclusion. Terms such as “radiomics” and “segmentation” were combined with the term, “biomarker”, and various iterations of aspects of management, such as “prognostication” and “treatment response” and specific HPB tumors (e.g., “HCC” “PDAC”, etc.).
DEFINING RADIOMICS
Recently, the field of radiomics has burgeoned and shown promise as a potential tool for early diagnosis, tumor characterization, and prognostication[19]. This involves extraction of high-dimensional data from images and providing feature data for quantitative description of lesions[20]. Radiographic images contain a number of quantifiable features that may be mined and analyzed to offer insights into disease processes[19]. The general radiomics workflow can be broadly summarized into four main steps: image acquisition, region of interest (ROI) segmentation, feature extraction, and analysis [Figure 1][21]. Image segmentation consists of the delineation of the boundaries of a ROI, such as a tumor and adjacent anatomical structures. This step can be done manually, semi-automatically, or fully automatically with deep learning algorithms. Manual segmentation and semi-automatic segmentation, though time-consuming, have been the most encountered methods of segmentation in current literature[22]. While these methods of segmentation are prone to introducing inter-observer biases stemming from inconsistencies in the delineation of the boundaries of ROIs, prior studies have uncovered conflicting results on the influence this actually has on radiomics features[21,23]. Once ROIs have been segmented, the radiomic features are extracted via the conversion of imaging data into quantifiable features, such as signal intensity, shape, texture, and higher-order features. Signal intensity features are obtained through analysis of histograms of individual voxel signal intensities and offer insights into the central tendency of the distribution. Shape and texture features are both calculated in three dimensions by considering the correlation of signal intensities of surrounding voxels. Higher-order features may also be extracted following the application of secondary wavelet or Gaussian filters[22]. The number of features extracted through these processes can significantly vary depending on filter and software specifications. Overfitting of the model may occur in cases where a high number of features are coupled with a low number of cases in a classification task. The next step of the workflow, feature selection, mitigates this risk by selecting relevant features using techniques such as random forest algorithms and by excluding non-reproducible features. Relevant features may then undergo subsequent analysis using machine learning algorithms.
APPLICATION OF RADIOMICS IN PANCREATIC CANCER
Pancreatic cancer is one of the leading causes of cancer-related deaths globally, with an estimated 5-year survival of approximately 12%[24]. As compared to other malignancies, the incidence of PC is on the rise while the outcomes remain poor. Over the last four decades, minimal improvement in survival has been observed, the only major development being the introduction of multiagent systemic therapies in the last decade[25]. The two key challenges that are faced in the management of these patients are the lack of screening tools, resulting in a delay in diagnosis and limited biomarkers that can help guide management in terms of selection, duration, and sequencing of therapeutics[26]. Oncological resection in conjunction with systemic therapy provides the best chance at cure.
A majority of patients are asymptomatic or present with non-specific symptoms such as abdominal pain and weight loss. Implementation of screening via magnetic resonance imaging (MRI) and endoscopic ultrasound (EUS) has shown promise in high-risk populations; however, implementing them across the entire population is not feasible due to the relatively low incidence of disease. Upon suspicion of disease, computed tomography (CT) coupled with EUS with fine needle biopsy can diagnose the disease. Given current approaches, only 20% of patients are diagnosed with resectable disease, and therefore, better screening tools are required. In terms of therapeutic selection, the only clinically available biomarker is carbohydrate antigen 19-9 (CA19-9), but it has several limitations. Firstly, it can be elevated in non-neoplastic diseases such as pancreatitis and biliary obstruction. Second, approximately 20% of patients are non-producers, rendering its use to estimate tumor biology infeasible. Third, biomarkers to predict sensitivity or resistance to systemic therapies are not available. As a result, treatment response can only be estimated several months into the administration of these therapies when the disease has typically progressed to an incurable stage.
As described earlier, radiomic features represent tumor characteristics and can be applied to various aspects of the management of pancreatic cancer, which are described as follows.
Diagnosis and surgical resectability
Early diagnosis of disease presents one of the greatest challenges in the management of pancreatic cancer. Radiomics has shown to be a promising tool for the differentiation of normal pancreatic tissue from pancreatic cancer. Application of radiomics has demonstrated strong discriminatory ability with the reported AUC [area under the receiver operating characteristic (ROC) curve] of 0.79-0.99[27-29]. Beyond normal pancreatic parenchyma, radiomics has also been employed in differentiating pancreatic cancer from various mimicking lesions, most notably autoimmune pancreatitis (AIP). Studies of CT-based radiomic features have demonstrated an accuracy of 85%-95.2% in differentiating AIP from PC[30-34]. Similar studies have been performed to differentiate PNETs from PDACs and SPT, achieving AUCs ranging from 0.86-0.99[35-38]. Of note, these studies included atypical hypovascular NF-PNETs, which more closely mimic PDAC than typical PNETs, and radiomics analysis outperformed clinic-radiological factors[36,37,39,40]. Radiomics combined with machine learning methods would potentially result in the development of tools that will allow for radiomics-based screening for asymptomatic pancreatic cancer. Though the sensitivity of CT in the detection of PDAC ranges from 76%-96%, early CT findings of PDAC, such as tumoral heterogeneity and loss of fatty marbling, can be particularly subtle and may be missed even by experienced radiologists[28]. Radiomics poses an avenue for quantitative analysis and detection of these changes. Radiomics analysis may also autonomously run in the background of scans and automate the process of screening[41]. This can effectively enable every abdominal CT scan, regardless of indication, to be used to screen for PDAC. The improved quantitative analysis of images combined with an increase in the sheer volume of scans being screened through this approach makes radiomics a suitable tool for screening for PDAC. Through this, it may be possible to detect disease at an earlier stage when a larger proportion of patients are amenable to surgical resection.
Pancreatic cysts present a diagnostic challenge and comprise a heterogeneous group of lesions with biological behavior varying between benign indolent lesions and a propensity for progression to invasive cancer. Radiomics has been applied to differentiate cyst types. In one of these studies, the authors were able to differentiate serous cystadenomas and pancreatic cystic lesions with an AUC of 0.77 based on 22 radiomic features, which outperformed clinical and standard imaging features (AUC: 0.71)[42]. In another study, Xie et al. were able to differentiate macrocystic SCNs and MCNs with an AUC of 0.99[43]. Available literature on studies applying radiomics to pancreatic cystic lesions was summarized by Machicado et al., who reported AUCs between 0.77 and 0.99 in differentiating different cyst types[44]. Mucinous cystic lesions of the pancreas are precursors to pancreatic cancer, but not all of these patients will go on to develop cancer[10]. Therefore, risk stratification is essential if we are to resect lesions prior to their progression to cancer while avoiding surgery in patients with benign lesions given the high morbidity and mortality associated with these procedures. Radiomics has been applied to the characterization of these cysts and has shown an accuracy of 84% in distinguishing between common types of pancreatic cysts[45].
Currently, work is being performed on assessing the utility of radiomics in detecting high-grade dysplasia in these lesions and predicting the risk of progression to pancreatic cancer. Tobaly et al. trained a radiomics model based on preoperative CT to differentiate low-grade dysplasia, high-grade dysplasia, and invasive cancer in patients with IPMN and demonstrated an AUC of 0.84 and 0.71 on internal and external validation, respectively[46]. Similarly, Polk et al. combined radiomics with conventional variables (thickened and enhanced cyst wall and enhanced mural nodule) and reported an AUC of 0.93 (95%CI: 0.85-1.0) in differentiating low-grade dysplasia and high-grade dysplasia or invasive cancer[47]. If these tools are developed, we will be able to accurately screen high-risk patients and recommend appropriate care.
Radiomic-based analysis could also improve surgical planning. Determination of resectability and the likelihood of margin negative resections, particularly in the setting of neoadjuvant therapy, is challenging, and the ability of conventional pancreas protocol CT (PPCT) to determine this remains low[48,49]. This is driven by the fact that it is difficult to differentiate dead tissue and viable tumors on a PPCT. Since radiomics provides a greater deal of information regarding the tissue in the region of interest, studies have applied radiomics to detect the presence of vascular invasion and predict positive resection margins and demonstrated improved prediction compared to conventional PPCT[50-52]. Recently, Schlanger et al. systematically reviewed studies employing artificial intelligence and machine learning across two categories: preoperative diagnosis and patient evolution; five studies focused on the prediction of complications[53]. Two of these studies developed imaging-based models, utilizing CT texture features and MRI-based features, respectively, to predict postoperative pancreatic fistula[54,55]. Capretti et al. similarly harnessed 100 preoperative CT scans to develop a model to predict clinically relevant postoperative pancreatic fistula with an AUC of 08.07, which was also able to predict postoperative overall complications and length of stays with AUCs of 0.690 and 0.709[56].
Characterizing tumor biology
One of the most promising applications of radiomics has been its ability to noninvasively assess and characterize tumor pathology. Considerable work has been done in correlating tumor grade with CT and MRI radiomic features. The majority of current work has been conducted on radiomics-guided grading in pancreatic neuroendocrine tumors and has found a remarkable predictive ability for radiomic analysis to preoperatively distinguish WHO grade 1 from WHO grade 2 or 3 lesions (AUC: 0.736-0.902)[57-61]. Similar results have been reported for pancreatic cancer (AUC: 0.91-0.994)[62,63]. Radiomic features have been shown to be able to accurately determine the preoperative risk of lymph node and liver metastases and superior mesenteric vein and portal vein invasion in pancreatic cancer with an AUC of 0.841-0.912[51,64-67]. Molecular analysis can allow for personalized approaches to the management of pancreatic cancer; however, it is currently limited by cost and the need for invasive procedures to acquire tissue[68]. Radiogenomics is an area of great interest where radiomic features can be used to determine the mutational profile of these lesions. Current studies on radiogenomics in pancreatic cancer have identified key radiomic features that are highly predictive of genetic alterations in SMAD4, KRAS and p53, HNF1a, and KRT81 status[69-72].
Predicting treatment response and prognostication
As discussed earlier, real-time assessment of treatment response is essential in the management of pancreatic cancer. However, currently available clinical tools fail to provide accurate assessment, resulting in delays in tailoring of therapy that enables us to derive maximum benefit from the administered therapies while minimizing toxicity. In this regard, studies have assessed radiomic features in a longitudinal manner across chemotherapy, known as delta radiomics[73]. These studies have reported a strong association between delta radiomics and treatment response gauged by changes in CA19-9, RECIST criteria, and grade of response observed on histopathological examination of the surgical specimen[74-79]. However, current studies are limited by the heterogeneity of the radiomic features found to be significantly associated with treatment response, the small sample size of the study population, and the single institution nature of these studies[73]. Future studies must consolidate current evidence by analyzing the various radiomic signatures used across the studies to create a core set of validated radiomics signatures that can be broadly translated to clinical practice. Lastly, radiomics has shown promise in prognostication. Radiomic models based on features extracted from pretreatment scans have been shown to be highly predictive of overall survival, local control, and recurrence and have outperformed current clinical prediction models with an AUC of 0.78-0.92[80-83].
APPLICATION OF RADIOMICS IN HEPATOBILIARY MALIGNANCIES
Diagnosis and surgical resectability
Unlike most solid cancers, the diagnosis of HCC & intrahepatic cholangiocarcinoma (ICC) can be established without biopsy confirmation[84]. As a result, the role of noninvasive imaging is of particular importance in the diagnostic workflow of these malignancies. While HCC most often presents with highly characteristic and specific imaging findings, it can occasionally be challenging to discern definitively on imaging from other mimicking hypervascular lesions such as hemangiomas, adenomas, and focal nodular hyperplasia (FNH)[85,86]. Radiomic-guided studies have demonstrated efficacy in differentiating benign liver lesions from malignant ones with an AUC of differentiating FNH from HCC ranging from 0.917 to 0.971, an AUC for differentiating hemangiomas from HCC of 0.83-0.96 and a sensitivity and specificity for accurately classifying adenoma 0.80-0.93/0.78-0.93[87-91]. Another study distinguished combined hepatocellular cholangiocarcinoma (C-HCC) from HCC with an AUC of 0.79-0.81 and outperformed current diagnostic criteria when used in conjunction to differentiate intrahepatic cholangiocarcinoma from HCC[92]. Radiomic features have also been applied to distinguish primary and metastatic liver tumors, with one study reporting an accuracy of 83% in differentiating metastatic liver disease from primary tumors[93]. Differentiating neoplastic portal vein involvement and thrombosis from benign portal vein thrombosis is also essential in determining the underlying HCC’s resectability. Canellas et al. were able to reliably distinguish neoplastic and bland thrombi using radiomics signatures based on thrombus density values[94]. Moreover, microinvasion, encompassing infiltration of portal vein, hepatic vein, or bile duct, is considered a marker of poor prognosis after hepatic resection and transplantation[95]. Several studies have also demonstrated promising results of select CT and U/S-based radiomics signatures in accurately predicting the presence of microvascular tumor invasion[96-99]. For ICC, in a study of 203 cases, radiomics-based models demonstrated the ability to predict futile resections with greater AUC (0.804) than traditional clinical risk factor-based models (AUC: 0.590)[100].
Characterization of tumor biology
Traditional biopsies are restricted to the small sample of tumor that is biopsied, which may not be representative of the tumor at large[101,102]. With radiomics-based tumor grading, the entirety of the tumor is evaluated when being graded, thus providing physicians with greater certainty regarding tumor characteristics[103]. Applications of radiomics in this regard have demonstrated promise, with studies reporting that radiomics signatures strongly correlate with pathological grade of HCCs, allowing for rapid, noninvasive tumor grade determination[104]. Subsequent research has since further correlated radiomic signatures with tumor Ki-67 status, demonstrating the utility of radiomics in assessing HCC proliferation indices entirely noninvasively[105].
Treatment selection
Liver transplantation and liver resection represent potentially curative options for early-stage HCC; however, most patients present with later-stage HCC, rendering them ineligible for these options. As such, physicians and patients must choose from a variety of locoregional therapies, including radiofrequency ablation (RFA), transarterial chemoembolization (TACE), and transarterial radioembolization (TARE), all of which have particular advantages and disadvantages[106,107]. In recent years, there have been investigational efforts to harness radiomics to aid in this challenging clinical decision-making process. For instance, one group employed a DL-based radiomics strategy to develop nomograms for predicting progression-free survival among HCC patients undergoing liver resection or RFA (c-index = 0.726 for RFA, 0.741 for resection)[108]. To identify future candidates for RFA, another group calculated a radiomics signature based on textural features extracted from patients with significantly longer progression-free survival after RFA
Prognostication
Multiple studies have reported the value of applying radiomics to predict outcomes in hepatobiliary diseases. These studies demonstrated that the addition of radiomics to conventional clinical variables increases the accuracy of predicting early recurrence of disease, disease-specific recurrence, and long-term mortality as compared to clinical variables alone with AUCs ranging from 0.59 to 0.91[107,115,116]. For comparison, a prognostic nomogram incorporating age, N stage, M stage, tumor size, and surgery yielded AUCs of 0.909 and 0.890 for predicting 1-year and 5-year survival, respectively[117]. Similar studies have demonstrated certain radiomics features to accurately and independently predict post-surgical overall survival[118,119]. Seeing as HCC recurrence rates range from 25% after liver transplantation to over 70% after liver resection or RFA[120], numerous studies investigating the potential of radiomics in predicting recurrence risk after various treatments are also underway. In a multi-institutional study, based on contrast-enhanced computed tomography (CECT) analysis, preoperative and postoperative ML-based radiomics models were developed to predict HCC recurrence after liver resection, and both exhibited better prognostic performance compared to the current staging system (c-index = 0.733-0.801)[121]. Another study used CECT images from 184 patients to derive radiomics signatures of risk-straying patients based on their recurrence-free survival after RFA (c-index = 0.755)[122]. Other groups have also utilized DCE-MRI to develop radiomics models; for instance, one study compared the prognostic performance of radiomic scores for the tumor region, perilesional region, non-tumoral region, which independently had the highest prognostic score (c-index = 0.72); the combined score of the three regions yielded a c-index of 0.83[123]. Focusing on patients with unresectable disease, a separate study group used preoperative multiparameter MRI (mp-MRI) to create a radiomics model to predict early progression after TACE (AUC: 0.800)[106]. Furthermore, studies suggest that models combining radiomic and clinical input may provide the best performance. For instance, one study established a pretreatment CT-based radiomics model for overall survival after TACE and reported that a composite model was more predictive than a radiomics or clinical signature alone (HR: 19.88, 95%CI: 6.37-92.02, P < 0.001)[106].
Similar efforts to estimate treatment response and survival for CCA, which remains a highly lethal hepatobiliary malignancy, have been conducted. A number of studies have radiomics-based models to predict lymph node metastasis (LNM) in ICC patients with validation cohort AUCs ranging from 0.80 to 0.89[124-126]. Two studies have developed radiomics-based models for preoperative prediction of microvascular invasion (MVI)[127,128]. In another study focusing on LMN in ECC, an MRI-based radiomics model achieved an AUC of 0.9 in predicting LNM[129]. Other researchers have explored recurrence after surgery with implications for informing surveillance schedules and any adjuvant therapy. For example, one group employed random forest and logistic algorithms to develop clinical, radiomic, and combined models; the radiomics model had higher AUCs (sensitivity 0.846, specificity 0.771 in the validation cohort) than the clinical model and comparable AUCs to the combined model[100].
Altogether, current literature underscores that the incorporation of radiomics may enhance models to predict response to various treatments, thereby guiding appropriate treatment selection and sequencing. In facilitating more informed clinical decision-making, radiomics models will hopefully help improve patient outcomes for hepatobiliary cancers.
CURRENT PITFALLS
Despite promising results, the field of radiomics is still in its relative infancy. As of now, there are still certain critical limitations across the majority of literature on radiomics in hepatobiliary and pancreatic diseases, which need to be addressed prior to its clinical integration [Table 1].
Current limitations of radiomics
No. | Limitations |
1 | Limited validation of radiomics models |
2 | Segmentation required with most literature based on manual segmentation techniques (1) Operator dependent (2) Time intensive (3) Introduces inherent variation that radiomic features may be sensitive to |
3 | Lack of standardization in the workflow of radiomics |
4 | Radiomics features are sensitive to variations in imaging protocols, which limits multicentric generalization |
First, a vast majority of existing literature on radiomics comprises retrospective single-institution series with relatively small sample sizes. Currently, a majority of these radiomics-based models have not been validated in other datasets and are therefore not generalizable. Second, prior to the extraction of radiomic features from images, the areas of interest within those images must be delineated, a process known as segmentation[22]. Current segmentation methods are either manual, semi-automated or fully automated. Most literature on radiomics is based on manually segmented datasets. Manual segmentation introduces human error and is highly operator-dependent[22]. In the case of hepatobiliary and pancreatic malignancies, particularly pancreatic cancer, these tumors have irregular boundaries, which makes segmentation even more difficult. Although variations in segmentation methods have been linked to introducing specific differences in radiomic signatures, no current consensus exists on the segmentation techniques. Deep-learning-based segmentation has shown promise in bridging this gap; however, it depends on the accumulation of large datasets to train these models. Work is underway; however, this has not yet been achieved. Additionally, manual segmentation is time-intensive and requires oversight by trained radiologists, thus hampering the feasibility of using it in the clinical setting. Third, in order to eliminate bias, mitigate confounding factors, and encourage reproducibility, the radiomics workflow of studies should be scrutinized and entirely standardized. Many current studies have ambiguous descriptions regarding their process of feature extraction algorithms, mathematical definitions, inconsistencies in feature nomenclature and pre-processing methodology[130]. This has been the source of skepticism from clinicians who perceive the radiomics model as a black box that generates satisfying clinical prediction results for any given clinical outcome[130]. Moving forwards, a clear and transparent description of all these processes, alongside any feature reduction or exclusion, should be attempted. Lastly, radiomic features have been demonstrated to be sensitive to variations in image acquisition protocols[22,131]. Even variations in the time at which contrast-enhanced images are captured following the administration of contrast have been shown to significantly affect the radiomic features of acquired images[132]. Due to this, imaging protocols must be standardized across all centers evaluating radiomics. Efforts are underway to address these shortcomings, one example of which is the introduction of the radiomics quality score (RQS), a generalizable tool to assess the quality of radiomic studies, which is now being integrated into a majority of studies in the field[133].
FUTURE DIRECTIONS AND CLINICAL INTEGRATION
Despite limitations, current results of radiomics-based studies are encouraging and denote a promising future for the field. As the radiomics workflow becomes more standardized and the radiomics process becomes more easily reproducible, significant clinical implications can be expected in the following domains.
As a screening tool, radiomics has already demonstrated significant potential in differentiating normal tissue from malignancy. With currently ongoing work on automated deep learning-based radiomics workflow, it can be expected that tools that can accurately screen for hepatobiliary and pancreatic malignancies will be developed. Upon validation, these can then be made available for widespread clinical integration. This tool could be run on radiographic images to autonomously highlight suspicious areas of interest and bring them to the attention of a reading radiologist, providing a probability assessment of potential early malignancy.
For diagnostics, current diagnostic criteria consist of varying combinations of pathology- and radiology-based input to reach a definitive diagnosis. Both these methods, however, are limited by their current accuracy in differentiating lesions and human error in the workflow. Radiomics has already demonstrated the potential to accurately diagnose malignancies through their unique radiomic footprints. This is of particular importance in pancreatic lesions where there is a significant overlap in radiological features between these lesions. Additionally, radiomics has demonstrated the ability to noninvasively predict tumor characteristics with high accuracy, including tumor grade and the presence of nodal disease. In particular, for tumors that demonstrated intertumoral heterogeneity, such as pancreatic neuroendocrine tumors, pathological assessment is limited to the tissue obtained on biopsies and might fail to provide information about the whole lesion[134,135]. Radiomics allows for “virtual biopsies” that can overcome this by capturing the spatial heterogeneity of the entire tumor, enabling optimal characterization. Furthermore, the noninvasive nature of radiomics can result in repeat assessment at varying intervals for patients who are on surveillance. Another interesting application in tumor characterization is the emerging field of radiogenomics. Albeit limited, the literature available on this has suggested that radiomics can be used to genetically characterize these tumors. As this field develops, radiomics has the potential to allow for accurate diagnostics and tumor characterization that is superior to the current diagnostic modalities.
Radiomics has also demonstrated potential in predicting response to systemic therapies noninvasively. Current biomarkers fail to accurately predict response to administered therapies, resulting in delays in tailoring of therapies in a timely manner. If radiomics-based assessment of treatment response were possible, it would allow for timely modification in systemic therapy, thus maximizing the chance for complete or near complete treatment response and improved survival. In terms of surgical planning, radiomics has demonstrated the ability to predict the presence of vascular invasion more accurately than experienced radiologists. Lastly, radiomics has shown promise in prognostication via accurate prediction of postoperative complications, and recurrence-free and overall survival. Accurate prognostication is essential not only in guiding management, as previously discussed, but also in managing patient expectations through the course of their care. Radiomics-based prognostication has outperformed a variety of existing prognostic factors. In studies where radiomics has not independently outperformed existing factors, the addition of radiomics to established factors enhanced the accuracy of the existing models. While it is unlikely that radiomics models will ever independently be used to prognosticate patients, it is very likely that models combining radiomic features and clinical factors will be used across all metrics of prognostication in the future.
CONCLUSIONS
In conclusion, the field of radiomics is advancing rapidly and has shown promise as a tool for early detection, tumor characterization, therapeutic selection, and prognostication in hepatobiliary and pancreatic malignancies. Despite its shortcomings, we believe that with improvements and automation of segmentation techniques, optimization of radiomic analyses, and introduction of standardized guidelines for research on radiomics, the tools developed using this technology will become more robust. Clinical application of these tools will provide precision in the management of these patients, resulting in improvement in patient outcomes.
DECLARATIONS
Authors’ contributions
Made substantial contributions to the conception and design of the study and performed data analysis and interpretation: Grewal M, Ahmed T, Javed AA
Performed data acquisition, as well as providing administrative, technical, and material support: Grewal M, Ahmed T, Javed AA
Availability of data and materials
Not applicable.
Financial support and sponsorship
None.
Conflicts of interest
All authors declared that there are no conflicts of interest.
Ethical approval and consent to participate
Not applicable.
Consent for publication
Not applicable.
Copyright
© The Author(s) 2023.
REFERENCES
1. Rawla P, Sunkara T, Gaduputi V. Epidemiology of pancreatic cancer: global trends, etiology and risk factors. World J Oncol 2019;10:10-27.
2. Bridgewater J, Lopes A, Wasan H, et al. Prognostic factors for progression-free and overall survival in advanced biliary tract cancer. Ann Oncol 2016;27:134-40.
3. Huang L, Jansen L, Balavarca Y, et al. Stratified survival of resected and overall pancreatic cancer patients in Europe and the USA in the early twenty-first century: a large, international population-based study. BMC Med 2018;16:125.
4. Brar G, Greten TF, Graubard BI, et al. Hepatocellular carcinoma survival by etiology: a SEER-medicare database analysis. Hepatol Commun 2020;4:1541-51.
5. Mittal S, El-Serag HB. Epidemiology of hepatocellular carcinoma: consider the population. J Clin Gastroenterol 2013;47 Suppl:S2-6.
6. Zhang L, Sanagapalli S, Stoita A. Challenges in diagnosis of pancreatic cancer. World J Gastroenterol 2018;24:2047-60.
7. Wang W, Wei C. Advances in the early diagnosis of hepatocellular carcinoma. Genes Dis 2020;7:308-19.
8. Kennedy T, Preczewski L, Stocker SJ, et al. Incidence of benign inflammatory disease in patients undergoing Whipple procedure for clinically suspected carcinoma: a single-institution experience. Am J Surg 2006;191:437-41.
9. Piñero F, Dirchwolf M, Pessôa MG. Biomarkers in hepatocellular carcinoma: diagnosis, prognosis and treatment response assessment. Cells 2020;9:1370.
10. Singhi AD, Koay EJ, Chari ST, Maitra A. Early detection of pancreatic cancer: opportunities and challenges. Gastroenterology 2019;156:2024-40.
11. Buchs NC, Chilcott M, Poletti PA, Buhler LH, Morel P. Vascular invasion in pancreatic cancer: Imaging modalities, preoperative diagnosis and surgical management. World J Gastroenterol 2010;16:818-31.
12. Rehders A, Stoecklein NH, Güray A, Riediger R, Alexander A, Knoefel WT. Vascular invasion in pancreatic cancer: tumor biology or tumor topography? Surgery 2012;152:S143-51.
13. Dayimu A, Di Lisio L, Anand S, et al. Clinical and biological markers predictive of treatment response associated with metastatic pancreatic adenocarcinoma. Br J Cancer 2023;128:1672-80.
14. Sturm N, Ettrich TJ, Perkhofer L. The impact of biomarkers in pancreatic ductal adenocarcinoma on diagnosis, surveillance and therapy. Cancers 2022;14:217.
15. Zhang WH, Wang WQ, Han X, et al. Advances on diagnostic biomarkers of pancreatic ductal adenocarcinoma: a systems biology perspective. Comput Struct Biotechnol J 2020;18:3606-14.
16. Herreros-Villanueva M, Bujanda L. Non-invasive biomarkers in pancreatic cancer diagnosis: what we need versus what we have. Ann Transl Med 2016;4:134.
17. Boilève A, Hilmi M, Delaye M, Tijeras-Raballand A, Neuzillet C. Biomarkers in hepatobiliary cancers: what is useful in clinical practice? Cancers 2021;13:2708.
19. Gillies RJ, Kinahan PE, Hricak H. Radiomics: images are more than pictures, they are data. Radiology 2016;278:563-77.
20. Karmazanovsky G, Gruzdev I, Tikhonova V, Kondratyev E, Revishvili A. Computed tomography-based radiomics approach in pancreatic tumors characterization. Radiol Med 2021;126:1388-95.
21. Mori M, Benedetti G, Partelli S, et al. Ct radiomic features of pancreatic neuroendocrine neoplasms (panNEN) are robust against delineation uncertainty. Phys Med 2019;57:41-6.
22. van Timmeren JE, Cester D, Tanadini-Lang S, Alkadhi H, Baessler B. Radiomics in medical imaging-“how-to” guide and critical reflection. Insights Imaging 2020;11:91.
23. Pavic M, Bogowicz M, Würms X, et al. Influence of inter-observer delineation variability on radiomics stability in different tumor sites. Acta Oncol 2018;57:1070-4.
24. Siegel RL, Miller KD, Wagle NS, Jemal A. Cancer statistics, 2023. CA Cancer J Clin 2023;73:17-48.
25. Habib JR, Kinny-Köster B, van Oosten F, et al. Periadventitial dissection of the superior mesenteric artery for locally advanced pancreatic cancer: surgical planning with the “halo sign” and “string sign”. Surgery 2021;169:1026-31.
26. Kinny-Köster B, Habib JR, Wolfgang CL, He J, Javed AA. Favorable tumor biology in locally advanced pancreatic cancer-beyond CA19-9. J Gastrointest Oncol 2021;12:2484-94.
27. Chen PT, Chang D, Yen H, et al. Radiomic features at CT can distinguish pancreatic cancer from noncancerous pancreas. Radiol Imaging Cancer 2021;3:e210010.
28. Chu LC, Park S, Kawamoto S, et al. Utility of CT radiomics features in differentiation of pancreatic ductal adenocarcinoma from normal pancreatic tissue. AJR Am J Roentgenol 2019;213:349-57.
29. Qiu JJ, Yin J, Qian W, et al. A novel multiresolution-statistical texture analysis architecture: radiomics-aided diagnosis of PDAC based on plain CT images. IEEE Trans Med Imaging 2021;40:12-25.
30. Park S, Chu LC, Hruban RH, et al. Differentiating autoimmune pancreatitis from pancreatic ductal adenocarcinoma with CT radiomics features. Diagn Interv Imaging 2020;101:555-64.
31. Ren S, Zhao R, Zhang J, et al. Diagnostic accuracy of unenhanced CT texture analysis to differentiate mass-forming pancreatitis from pancreatic ductal adenocarcinoma. Abdom Radiol 2020;45:1524-33.
32. Zhang Y, Cheng C, Liu Z, et al. Radiomics analysis for the differentiation of autoimmune pancreatitis and pancreatic ductal adenocarcinoma in 18F-FDG PET/CT. Med Phys 2019;46:4520-30.
33. Ziegelmayer S, Kaissis G, Harder F, et al. Deep convolutional neural network-assisted feature extraction for diagnostic discrimination and feature visualization in pancreatic ductal adenocarcinoma (PDAC) versus autoimmune pancreatitis (AIP). J Clin Med 2020;9:4013.
34. E L, Xu Y, Wu Z, et al. Differentiation of focal-type autoimmune pancreatitis from pancreatic ductal adenocarcinoma using radiomics based on multiphasic computed tomography. J Comput Assist Tomogr 2020;44:511-8.
35. Guo C, Zhuge X, Wang Q, et al. The differentiation of pancreatic neuroendocrine carcinoma from pancreatic ductal adenocarcinoma: the values of CT imaging features and texture analysis. Cancer Imaging 2018;18:37.
36. He M, Liu Z, Lin Y, et al. Differentiation of atypical non-functional pancreatic neuroendocrine tumor and pancreatic ductal adenocarcinoma using CT based radiomics. Eur J Radiol 2019;117:102-11.
37. Li J, Lu J, Liang P, et al. Differentiation of atypical pancreatic neuroendocrine tumors from pancreatic ductal adenocarcinomas: using whole-tumor CT texture analysis as quantitative biomarkers. Cancer Med 2018;7:4924-31.
38. Shi YJ, Zhu HT, Liu YL, et al. Radiomics analysis based on diffusion kurtosis imaging and T2 weighted imaging for differentiation of pancreatic neuroendocrine tumors from solid pseudopapillary tumors. Front Oncol 2020;10:1624.
39. Amedei A, Niccolai E, Prisco D. Pancreatic cancer: role of the immune system in cancer progression and vaccine-based immunotherapy. Hum Vaccin Immunother 2014;10:3354-68.
40. Jeon SK, Lee JM, Joo I, et al. Nonhypervascular pancreatic neuroendocrine tumors: differential diagnosis from pancreatic ductal adenocarcinomas at MR imaging-retrospective cross-sectional study. Radiology 2017;284:77-87.
41. Ahmed TM, Kawamoto S, Hruban RH, Fishman EK, Soyer P, Chu LC. A primer on artificial intelligence in pancreatic imaging. Diagn Interv Imaging 2023;104:435-47.
42. Wei R, Lin K, Yan W, et al. Computer-aided diagnosis of pancreas serous cystic neoplasms: a radiomics method on preoperative MDCT images. Technol Cancer Res Treat 2019;18:1533033818824339.
43. Xie H, Ma S, Guo X, Zhang X, Wang X. Preoperative differentiation of pancreatic mucinous cystic neoplasm from macrocystic serous cystic adenoma using radiomics: preliminary findings and comparison with radiological model. Eur J Radiol 2020;122:108747.
44. Machicado JD, Koay EJ, Krishna SG. Radiomics for the diagnosis and differentiation of pancreatic cystic lesions. Diagnostics 2020;10:505.
45. Dmitriev K, Kaufman AE, Javed AA, et al. Classification of pancreatic cysts in computed tomography images using a random forest and convolutional neural network ensemble. In: Descoteaux M, Maier-hein L, Franz A, Jannin P, Collins DL, Duchesne S, editors. Medical image computing and computer assisted intervention - MICCAI 2017. Cham: Springer International Publishing; 2017. p. 150-8.
46. Tobaly D, Santinha J, Sartoris R, et al. CT-based radiomics analysis to predict malignancy in patients with intraductal papillary mucinous neoplasm (IPMN) of the pancreas. Cancers 2020;12:3089.
47. Polk SL, Choi JW, McGettigan MJ, et al. Multiphase computed tomography radiomics of pancreatic intraductal papillary mucinous neoplasms to predict malignancy. World J Gastroenterol 2020;26:3458-71.
48. Hong SB, Lee SS, Kim JH, et al. Pancreatic cancer CT: prediction of resectability according to NCCN criteria. Radiology 2018;289:710-8.
49. Zins M, Matos C, Cassinotto C. Pancreatic adenocarcinoma staging in the era of preoperative chemotherapy and radiation therapy. Radiology 2018;287:374-90.
50. Bian Y, Jiang H, Ma C, et al. Performance of CT-based radiomics in diagnosis of superior mesenteric vein resection margin in patients with pancreatic head cancer. Abdom Radiol 2020;45:759-73.
51. Chen F, Zhou Y, Qi X, et al. Radiomics-assisted presurgical prediction for surgical portal vein-superior mesenteric vein invasion in pancreatic ductal adenocarcinoma. Front Oncol 2020;10:523543.
52. Rigiroli F, Hoye J, Lerebours R, et al. CT radiomic features of superior mesenteric artery involvement in pancreatic ductal adenocarcinoma: a pilot study. Radiology 2021;301:610-22.
53. Schlanger D, Graur F, Popa C, Moiș E, Al Hajjar N. The role of artificial intelligence in pancreatic surgery: a systematic review. Updates Surg 2022;74:417-29.
54. Kambakamba P, Mannil M, Herrera PE, et al. The potential of machine learning to predict postoperative pancreatic fistula based on preoperative, non-contrast-enhanced CT: a proof-of-principle study. Surgery 2020;167:448-54.
55. Skawran SM, Kambakamba P, Baessler B, et al. Can magnetic resonance imaging radiomics of the pancreas predict postoperative pancreatic fistula? Eur J Radiol 2021;140:109733.
56. Capretti G, Bonifacio C, De Palma C, et al. A machine learning risk model based on preoperative computed tomography scan to predict postoperative outcomes after pancreatoduodenectomy. Updates Surg 2022;74:235-43.
57. Bian Y, Jiang H, Ma C, et al. CT-based radiomics score for distinguishing between grade 1 and grade 2 nonfunctioning pancreatic neuroendocrine tumors. AJR Am J Roentgenol 2020;215:852-63.
58. Bian Y, Li J, Cao K, et al. Magnetic resonance imaging radiomic analysis can preoperatively predict G1 and G2/3 grades in patients with NF-pNETs. Abdom Radiol 2021;46:667-80.
59. Bian Y, Zhao Z, Jiang H, et al. Noncontrast radiomics approach for predicting grades of nonfunctional pancreatic neuroendocrine tumors. J Magn Reson Imaging 2020;52:1124-36.
60. Choi TW, Kim JH, Yu MH, Park SJ, Han JK. Pancreatic neuroendocrine tumor: prediction of the tumor grade using CT findings and computerized texture analysis. Acta Radiol 2018;59:383-92.
61. Liang W, Yang P, Huang R, et al. A combined nomogram model to preoperatively predict histologic grade in pancreatic neuroendocrine tumors. Clin Cancer Res 2019;25:584-94.
62. Ren S, Zhao R, Cui W, et al. Computed tomography-based radiomics signature for the preoperative differentiation of pancreatic adenosquamous carcinoma from pancreatic ductal adenocarcinoma. Front Oncol 2020;10:1618.
63. Xing H, Hao Z, Zhu W, et al. Preoperative prediction of pathological grade in pancreatic ductal adenocarcinoma based on 18F-FDG PET/CT radiomics. EJNMMI Res 2021;11:19.
64. Bian Y, Guo S, Jiang H, et al. Relationship between radiomics and risk of lymph node metastasis in pancreatic ductal adenocarcinoma. Pancreas 2019;48:1195-203.
65. Gao J, Han F, Jin Y, Wang X, Zhang J. A radiomics nomogram for the preoperative prediction of lymph node metastasis in pancreatic ductal adenocarcinoma. Front Oncol 2020;10:1654.
66. Li K, Yao Q, Xiao J, et al. Contrast-enhanced CT radiomics for predicting lymph node metastasis in pancreatic ductal adenocarcinoma: a pilot study. Cancer Imaging 2020;20:12.
67. Liu P, Gu Q, Hu X, et al. Applying a radiomics-based strategy to preoperatively predict lymph node metastasis in the resectable pancreatic ductal adenocarcinoma. J Xray Sci Technol 2020;28:1113-21.
68. Lo Gullo R, Daimiel I, Morris EA, Pinker K. Combining molecular and imaging metrics in cancer: radiogenomics. Insights Imaging 2020;11:1.
69. Attiyeh MA, Chakraborty J, McIntyre CA, et al. CT radiomics associations with genotype and stromal content in pancreatic ductal adenocarcinoma. Abdom Radiol 2019;44:3148-57.
70. Iwatate Y, Hoshino I, Yokota H, et al. Radiogenomics for predicting p53 status, PD-L1 expression, and prognosis with machine learning in pancreatic cancer. Br J Cancer 2020;123:1253-61.
71. Kaissis GA, Ziegelmayer S, Lohöfer FK, et al. Image-based molecular phenotyping of pancreatic ductal adenocarcinoma. J Clin Med 2020;9:724.
72. Lim CH, Cho YS, Choi JY, et al. Imaging phenotype using 18F-fluorodeoxyglucose positron emission tomography-based radiomics and genetic alterations of pancreatic ductal adenocarcinoma. Eur J Nucl Med Mol Imaging 2020;47:2113-22.
73. Nardone V, Reginelli A, Grassi R, et al. Delta radiomics: a systematic review. Radiol Med 2021;126:1571-83.
74. Chen X, Oshima K, Schott D, et al. Assessment of treatment response during chemoradiation therapy for pancreatic cancer based on quantitative radiomic analysis of daily CTs: An exploratory study. PLoS One 2017;12:e0178961.
75. Cusumano D, Boldrini L, Yadav P, et al. Delta radiomics analysis for local control prediction in pancreatic cancer patients treated using magnetic resonance guided radiotherapy. Diagnostics 2021;11:72.
76. Nasief H, Hall W, Zheng C, et al. Improving treatment response prediction for chemoradiation therapy of pancreatic cancer using a combination of delta-radiomics and the clinical biomarker CA19-9. Front Oncol 2019;9:1464.
77. Nasief H, Zheng C, Schott D, et al. A machine learning based delta-radiomics process for early prediction of treatment response of pancreatic cancer. NPJ Precis Oncol 2019;3:25.
78. Simpson G, Spieler B, Dogan N, et al. Predictive value of 0.35 T magnetic resonance imaging radiomic features in stereotactic ablative body radiotherapy of pancreatic cancer: a pilot study. Med Phys 2020;47:3682-90.
79. Yue Y, Osipov A, Fraass B, et al. Identifying prognostic intratumor heterogeneity using pre- and post-radiotherapy 18F-FDG PET images for pancreatic cancer patients. J Gastrointest Oncol 2017;8:127-38.
80. Li X, Wan Y, Lou J, et al. Preoperative recurrence prediction in pancreatic ductal adenocarcinoma after radical resection using radiomics of diagnostic computed tomography. EClinicalMedicine 2022;43:101215.
81. Parr E, Du Q, Zhang C, et al. Radiomics-based outcome prediction for pancreatic cancer following stereotactic body radiotherapy. Cancers 2020;12:1051.
82. Tang TY, Li X, Zhang Q, et al. Development of a novel multiparametric MRI radiomic nomogram for preoperative evaluation of early recurrence in resectable pancreatic cancer. J Magn Reson Imaging 2020;52:231-45.
83. Wei M, Gu B, Song S, et al. A novel validated recurrence stratification system based on 18F-FDG PET/CT radiomics to guide surveillance after resection of pancreatic cancer. Front Oncol 2021;11:650266.
84. Marrero JA, Kulik LM, Sirlin CB, et al. Diagnosis, staging, and management of hepatocellular carcinoma: 2018 practice guidance by the American Association for the study of liver diseases. Hepatology 2018;68:723-50.
85. Joo I, Lee JM, Yoon JH. Imaging diagnosis of intrahepatic and perihilar cholangiocarcinoma: recent advances and challenges. Radiology 2018;288:7-13.
86. Potretzke TA, Tan BR, Doyle MB, Brunt EM, Heiken JP, Fowler KJ. Imaging features of biphenotypic primary liver carcinoma (hepatocholangiocarcinoma) and the potential to mimic hepatocellular carcinoma: LI-RADS analysis of CT and MRI features in 61 cases. AJR Am J Roentgenol 2016;207:25-31.
87. Gatos I, Tsantis S, Karamesini M, et al. Focal liver lesions segmentation and classification in nonenhanced T2-weighted MRI. Med Phys 2017;44:3695-705.
88. Jansen MJA, Kuijf HJ, Veldhuis WB, Wessels FJ, Viergever MA, Pluim JPW. Automatic classification of focal liver lesions based on MRI and risk factors. PLoS One 2019;14:e0217053.
89. Li Z, Mao Y, Huang W, et al. Texture-based classification of different single liver lesion based on SPAIR T2W MRI images. BMC Med Imaging 2017;17:42.
90. Nie P, Yang G, Guo J, et al. A CT-based radiomics nomogram for differentiation of focal nodular hyperplasia from hepatocellular carcinoma in the non-cirrhotic liver. Cancer Imaging 2020;20:20.
91. Wu J, Liu A, Cui J, Chen A, Song Q, Xie L. Radiomics-based classification of hepatocellular carcinoma and hepatic haemangioma on precontrast magnetic resonance images. BMC Med Imaging 2019;19:23.
92. Liu X, Khalvati F, Namdar K, et al. Can machine learning radiomics provide pre-operative differentiation of combined hepatocellular cholangiocarcinoma from hepatocellular carcinoma and cholangiocarcinoma to inform optimal treatment planning? Eur Radiol 2021;31:244-55.
93. Trivizakis E, Manikis GC, Nikiforaki K, et al. Extending 2-D convolutional neural networks to 3-D for advancing deep learning cancer classification with application to MRI liver tumor differentiation. IEEE J Biomed Health Inform 2019;23:923-30.
94. Canellas R, Mehrkhani F, Patino M, Kambadakone A, Sahani D. Characterization of portal vein thrombosis (neoplastic versus bland) on CT images using software-based texture analysis and thrombus density (Hounsfield Units). AJR Am J Roentgenol 2016;207:W81-7.
95. Santambrogio R, Barabino M, D’Alessandro V, et al. Micronvasive behaviour of single small hepatocellular carcinoma: which treatment? Updates Surg 2021;73:1359-69.
96. He M, Zhang P, Ma X, He B, Fang C, Jia F. Radiomic feature-based predictive model for microvascular invasion in patients with hepatocellular carcinoma. Front Oncol 2020;10:574228.
97. Ni M, Zhou X, Lv Q, et al. Radiomics models for diagnosing microvascular invasion in hepatocellular carcinoma: which model is the best model? Cancer Imaging 2019;19:60.
98. Qu C, Wang Q, Li C, et al. A radiomics model based on Gd-EOB-DTPA-enhanced MRI for the prediction of microvascular invasion in solitary hepatocellular carcinoma ≤ 5 cm. Front Oncol 2022;12:831795.
99. Zhang W, Yang R, Liang F, et al. Prediction of microvascular invasion in hepatocellular carcinoma with a multi-disciplinary team-like radiomics fusion model on dynamic contrast-enhanced computed tomography. Front Oncol 2021;11:660629.
100. Chu H, Liu Z, Liang W, et al. Radiomics using CT images for preoperative prediction of futile resection in intrahepatic cholangiocarcinoma. Eur Radiol 2021;31:2368-76.
101. Limkin EJ, Sun R, Dercle L, et al. Promises and challenges for the implementation of computational medical imaging (radiomics) in oncology. Ann Oncol 2017;28:1191-206.
102. Lopez A, Harada K, Mizrak Kaya D, Dong X, Song S, Ajani JA. Liquid biopsies in gastrointestinal malignancies: when is the big day? Expert Rev Anticancer Ther 2018;18:19-38.
103. Dalal V, Carmicheal J, Dhaliwal A, Jain M, Kaur S, Batra SK. Radiomics in stratification of pancreatic cystic lesions: machine learning in action. Cancer Lett 2020;469:228-37.
104. Wu M, Tan H, Gao F, et al. Predicting the grade of hepatocellular carcinoma based on non-contrast-enhanced MRI radiomics signature. Eur Radiol 2019;29:2802-11.
105. Ye Z, Jiang H, Chen J, et al. Texture analysis on gadoxetic acid enhanced-MRI for predicting Ki-67 status in hepatocellular carcinoma: a prospective study. Chin J Cancer Res 2019;31:806-17.
106. Yao S, Ye Z, Wei Y, Jiang HY, Song B. Radiomics in hepatocellular carcinoma: a state-of-the-art review. World J Gastrointest Oncol 2021;13:1599-615.
107. Miranda Magalhaes Santos JM, Clemente Oliveira B, Araujo-Filho JAB, et al. State-of-the-art in radiomics of hepatocellular carcinoma: a review of basic principles, applications, and limitations. Abdom Radiol 2020;45:342-53.
108. Castaldo A, De Lucia DR, Pontillo G, et al. State of the art in artificial intelligence and radiomics in hepatocellular carcinoma. Diagnostics 2021;11:1194.
109. Granata V, Fusco R, Belli A, et al. Conventional, functional and radiomics assessment for intrahepatic cholangiocarcinoma. Infect Agent Cancer 2022;17:13.
110. Suh SW, Lee KW, Lee JM, et al. Prediction of aggressiveness in early-stage hepatocellular carcinoma for selection of surgical resection. J Hepatol 2014;60:1219-24.
111. Kim J, Choi SJ, Lee SH, Lee HY, Park H. Predicting survival using pretreatment ct for patients with hepatocellular carcinoma treated with transarterial chemoembolization: comparison of models using radiomics. AJR Am J Roentgenol 2018;211:1026-34.
112. Reimer RP, Reimer P, Mahnken AH. Assessment of therapy response to transarterial radioembolization for liver metastases by means of post-treatment MRI-based texture analysis. Cardiovasc Intervent Radiol 2018;41:1545-56.
113. Chen S, Feng S, Wei J, et al. Pretreatment prediction of immunoscore in hepatocellular cancer: a radiomics-based clinical model based on Gd-EOB-DTPA-enhanced MRI imaging. Eur Radiol 2019;29:4177-87.
114. Sun R, Limkin EJ, Vakalopoulou M, et al. A radiomics approach to assess tumour-infiltrating CD8 cells and response to anti-PD-1 or anti-PD-L1 immunotherapy: an imaging biomarker, retrospective multicohort study. Lancet Oncol 2018;19:1180-91.
115. Zhou W, Zhang L, Wang K, et al. Malignancy characterization of hepatocellular carcinomas based on texture analysis of contrast-enhanced MR images. J Magn Reson Imaging 2017;45:1476-84.
116. Zhou Y, He L, Huang Y, et al. CT-based radiomics signature: a potential biomarker for preoperative prediction of early recurrence in hepatocellular carcinoma. Abdom Radiol 2017;42:1695-704.
117. Hu J, Wang Y, Deng L, et al. Development and validation of a nomogram for predicting the cancer-specific survival of fibrolamellar hepatocellular carcinoma patients. Updates Surg 2022;74:1589-99.
118. Liu Q, Li J, Liu F, et al. A radiomics nomogram for the prediction of overall survival in patients with hepatocellular carcinoma after hepatectomy. Cancer Imaging 2020;20:82.
119. Wang XH, Long LH, Cui Y, et al. MRI-based radiomics model for preoperative prediction of 5-year survival in patients with hepatocellular carcinoma. Br J Cancer 2020;122:978-85.
120. Tabrizian P, Jibara G, Shrager B, Schwartz M, Roayaie S. Recurrence of hepatocellular cancer after resection: patterns, treatments, and prognosis. Ann Surg 2015;261:947-55.
121. Ji GW, Zhu FP, Xu Q, et al. Machine-learning analysis of contrast-enhanced CT radiomics predicts recurrence of hepatocellular carcinoma after resection: a multi-institutional study. EBioMedicine 2019;50:156-65.
122. Yuan C, Wang Z, Gu D, et al. Prediction early recurrence of hepatocellular carcinoma eligible for curative ablation using a Radiomics nomogram. Cancer Imaging 2019;19:21.
123. Zhang Z, Chen J, Jiang H, et al. Gadoxetic acid-enhanced MRI radiomics signature: prediction of clinical outcome in hepatocellular carcinoma after surgical resection. Ann Transl Med 2020;8:870.
124. Ji GW, Zhang YD, Zhang H, et al. Biliary tract cancer at CT: a radiomics-based model to predict lymph node metastasis and survival outcomes. Radiology 2019;290:90-8.
125. Peng YT, Zhou CY, Lin P, et al. Preoperative ultrasound radiomics signatures for noninvasive evaluation of biological characteristics of intrahepatic cholangiocarcinoma. Acad Radiol 2020;27:785-97.
126. Xu L, Yang P, Liang W, et al. A radiomics approach based on support vector machine using MR images for preoperative lymph node status evaluation in intrahepatic cholangiocarcinoma. Theranostics 2019;9:5374-85.
127. Xiang F, Wei S, Liu X, Liang X, Yang L, Yan S. Radiomics analysis of contrast-enhanced CT for the preoperative prediction of microvascular invasion in mass-forming intrahepatic cholangiocarcinoma. Front Oncol 2021;11:774117.
128. Zhou Y, Zhou G, Zhang J, Xu C, Wang X, Xu P. Radiomics signature on dynamic contrast-enhanced MR images: a potential imaging biomarker for prediction of microvascular invasion in mass-forming intrahepatic cholangiocarcinoma. Eur Radiol 2021;31:6846-55.
129. Yang C, Huang M, Li S, et al. Radiomics model of magnetic resonance imaging for predicting pathological grading and lymph node metastases of extrahepatic cholangiocarcinoma. Cancer Lett 2020;470:1-7.
130. Liu Z, Wang S, Dong D, et al. The applications of radiomics in precision diagnosis and treatment of oncology: opportunities and challenges. Theranostics 2019;9:1303-22.
131. Zhao B. Understanding sources of variation to improve the reproducibility of radiomics. Front Oncol 2021;11:633176.
132. Fiz F, Costa G, Gennaro N, et al. Contrast administration impacts CT-based radiomics of colorectal liver metastases and non-tumoral liver parenchyma revealing the “Radiological” tumour microenvironment. Diagnostics 2021;11:1162.
133. Lambin P, Leijenaar RTH, Deist TM, et al. Radiomics: the bridge between medical imaging and personalized medicine. Nat Rev Clin Oncol 2017;14:749-62.
134. Junttila MR, de Sauvage FJ. Influence of tumour micro-environment heterogeneity on therapeutic response. Nature 2013;501:346-54.
Cite This Article
Export citation file: BibTeX | RIS
OAE Style
Grewal M, Ahmed T, Javed AA. Current state of radiomics in hepatobiliary and pancreatic malignancies. Art Int Surg 2023;3:217-32. http://dx.doi.org/10.20517/ais.2023.28
AMA Style
Grewal M, Ahmed T, Javed AA. Current state of radiomics in hepatobiliary and pancreatic malignancies. Artificial Intelligence Surgery. 2023; 3(4): 217-32. http://dx.doi.org/10.20517/ais.2023.28
Chicago/Turabian Style
Grewal, Mahip, Taha Ahmed, Ammar Asrar Javed. 2023. "Current state of radiomics in hepatobiliary and pancreatic malignancies" Artificial Intelligence Surgery. 3, no.4: 217-32. http://dx.doi.org/10.20517/ais.2023.28
ACS Style
Grewal, M.; Ahmed T.; Javed AA. Current state of radiomics in hepatobiliary and pancreatic malignancies. Art. Int. Surg. 2023, 3, 217-32. http://dx.doi.org/10.20517/ais.2023.28
About This Article
Special Issue
Copyright
Data & Comments
Data
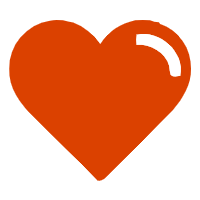

Comments
Comments must be written in English. Spam, offensive content, impersonation, and private information will not be permitted. If any comment is reported and identified as inappropriate content by OAE staff, the comment will be removed without notice. If you have any queries or need any help, please contact us at support@oaepublish.com.