Scoping review: autonomous endoscopic navigation
Abstract
This is a scoping review of artificial intelligence (AI) in flexible endoscopy (FE), encompassing both computer vision (CV) and autonomous actions (AA). While significant progress has been made in AI and FE, particularly in polyp detection and malignancy prediction, resulting in several available market products, these achievements only scratch the surface potential of AI in flexible endoscopy. Many doctors still do not fully grasp that contemporary robotic FE systems, which operate the endoscope through telemanipulation, represent the most basic autonomy level, specifically categorized as level 1. Although these console systems allow remote control, they lack the more sophisticated forms of autonomy. This manuscript aims to review the current examples of AI applications in FE and hopefully act as a stimulus for more advanced AA in FE.
Keywords
INTRODUCTION
Surgery today comprises various approaches, including traditional open surgery, minimally invasive surgery (MIS) such as endoscopic surgery and robotic surgery, and other interventional procedures such as interventional endoscopy, radiology, cardiology, and pulmonology. Specific names are used for endoscopic surgery in certain body cavities, such as laparoscopic (abdomen), thoracoscopic (thorax), or arthroscopic (joints). For the purposes of this manuscript, we will focus on flexible endoscopy (FE) conducted outside traditional open surgery or MIS, excluding rigid endoscopy or Natural orifice transluminal endoscopic surgery (NOTES). The current model of reusable FEs can limit availability and impact early detection. Manually controlled FE is constrained by the number of trained endoscopists and economic limitations that restrict the number of centers offering endoscopy. Furthermore, it could be argued that the root source of fatigue/learning curve is the antiquated controls of modern flexible scopes. This is highlighted by the fact that approximately 60% of the world’s population has no access to endoscopy. Artificial Intelligence (AI) has the potential to address these limitations[1].
AI has the potential to enhance the efficiency of endoscopy by integrating medical imaging and robotics. Examples of AI “in” endoscopy are the software-driven algorithms that utilize computer vision (CV) to detect and classify polyps into benign, premalignant and malignant lesions. CV aids in interpreting multispectral images beyond the visual spectrum of humans to better determine the depth of invasion in real time[2], whereas “Artificial intelligence endoscopy” (AIE) implies autonomous actions. AIE involves robotic assistance in navigating the endoscope within the targeted organ. Over the last decade, the emergence of AIE has showcased autonomous actions during endoscopy as one of the best avenues towards developing autonomy in other medical interventions. This progress might extend to more traditional surgeries such as open and MIS. Perhaps the most notable benefit of AIE would be its potential to address the scarcity of endoscopists throughout the world.
According to the World Health Organisation(WHO) in 2020, lung cancer (1.8 million deaths), colon and rectal cancer (916,000 deaths), liver cancer (830,000 deaths), and stomach cancer (769,000 deaths) are the most prevalent causes of cancer mortality[3]. For comparison, from 2019 to 2022, approximately 6 million lives were lost to COVID-2019. The advancement of autonomous endoscopic robots holds immense potential to significantly increase life-saving interventions each year. This is especially crucial given the presence of rigorous screening protocols that, if diligently implemented, could detect and treat diseases in their early stages, thereby reducing disease-related deaths[4].
The application of endoscopy such as bronchoscopy (visualizes airway), gastrointestinal endoscopy(visualizes gastrointestinal tract), and cystoscopy (visualize colon) have emerged as the primary approach for diagnosing and treating various hollow viscous pathologies, with the added benefit of minimal invasion and, therefore, shorter recovery times. The manual execution of these endoscopic procedures demands extensive training and expertise, involving careful manipulation and prolonged procedural times, which can lead to mental and physical fatigue and increase the risk of complications, for instance, perforation and bleeding. All of these could be ameliorated by autonomous endoscopes as they can essentially eliminate the fatigue and physical limitations of the operator. With the rapid development of robotics and sensor technology, interventional procedures have undergone remarkable changes with modern-day robotic-assisted procedures principally using telemanipulation or remote control to heighten precision and efficiency, but at the cost of reduced or no haptics (sense of touch)[5,6].
Currently, endoscopic navigation mainly relies on visualization from an external camera that uses depth estimation methods with variable reliability[7]. A study mentioned that Vision transformers (ViT) show a range of applications, including image processing, feature extraction without downsampling, and a global receptive field across all levels of the endoscopy. Furthermore, learning-based and model-less solutions for autonomous navigation of flexible endoscopes demonstrate greater efficacy by updating robot behavior with eye-in-hand visual configuration via estimate and learning approaches[7]. Robotic-assisted endoscopes, monocular visual guiding, a fiber Bragg grafting (FBG)-based proprioception unit, and learning-based model predictive control are all part of the proposed data-driven framework. The authors offer a data-driven framework for flexible endoscope autonomous navigation, concentrating on energy prediction and 3-D form planning to minimize excessive contact stresses on surrounding anatomy[7].
Some of the challenges in view of autonomous endoscopes include power limitations and adaptation to the varying shapes of the GI tract; however, innovative advancements continue to reshape endoscopic procedures, with the integration of robots and active propulsion mechanisms bringing transformative potential to these diagnostic and therapeutic interventions[8]. Robotics can enhance the present technique by offering a low-risk and, in certain situations, cost-effective alternative. Several firms have risen to the challenge in the previous two decades, bringing novel solutions to market. Clinical trials have shown that these devices have significant advantages over current technologies, such as lower pain, no need for sedation, and the potential for endoscopes to be disposable and thus less expensive, with the added benefit of eliminating the need to clean endoscopes, consequently minimizing the risk of cross-contamination. Certified agencies have certified compliance with health, safety, and environmental protection requirements[9].
Autonomous endoscopes may completely transform the field of minimally invasive interventional procedures by providing precision, efficiency, and enhanced patient outcomes. This technology may change the course of medicine by reducing the impact of human error and improving the abilities of healthcare workers via superior diagnostic and therapeutic care. This review aims to provide insights into autonomous endoscopic navigation, exploring its fundamental principles, key technological components, recent advancements, and diverse applications.
METHODOLOGY
This scoping review follows the Joanna Briggs Institute scoping review criteria and is reported using the Preferred Reporting Items for Systematic Review and Meta-Analyses extension for Scoping Reviews (PRISMA-ScR) checklist. No registration with Prospero was done because when we attempted to register for a systematic review, this request was denied. The research question was formulated using the PICOS format [Table 1].
Research question formulated using PICOS format
Population | Patients with various diseases and suspected precancerous and cancerous lesions, including respiratory, gastrointestinal, colorectal, and urological |
Intervention | Autonomous endoscopes using artificial intelligence, robotics, and medical imaging for reliability, accuracy, and meticulousness in diagnosis and treatment |
Comparison | Traditional endoscopic techniques and manual endoscopic surgeries without endoscopic autonomy |
Outcome | · Enhanced detection of benign and. malignant lesions · Minimized false positives and negatives and improved diagnostic accuracy · Reduction in missed adenomas and polyps · Reducing morbidity and mortality · Improving patient outcomes · Reduced need for longer learning curves and surgeon-specific training · Potential for atraumatic autonomous exploration of anatomical features · Higher detection rate for small and proliferative polyps · Reduced additional surgery after endoscopic resection · Enhanced efficiency and safety in soft tissue surgery |
Study design | Cohort studies, cross-sectional studies, case-control studies, randomized control trials reviews and editorials |
Search strategy
A literature search was performed and databases were searched from PUBMED & Google Scholar. An initial search was conducted in PUBMED with the following strategy: “Autonomous endoscopy” OR “Artificial Intelligence endoscopy” OR “Autonomous colonoscopy” OR “Autonomous bronchoscopy” OR “Autonomous gastroscopy” OR “Autonomous cystoscopy” OR “Robotic endoscopy” OR “Navigation endoscopy”. The title and abstract were used to find index phrases and keywords, which were then included in the search strategy.
Eligibility criteria
The search only includes peer-reviewed journal papers published in English between 2013 and 2023. The older articles written before 2013 were not included in this article because we were concentrating on the most current advancements in autonomous endoscopes. Only original articles from investigations such as randomized controlled trials, observational studies, case-control studies, and editorials were included in the review. Case reports and series were not included. Articles that focused more on the use of robotic surgery in various types of operations were also excluded because we wanted to concentrate more on the endoscopic approach. However, a brief discussion of the applications of the robotic endoscopic method to surgery was included.
Data items, synthesis of results
Each of the texts was screened for data related to types of endoscopes and applications. AI algorithms and techniques, success rate, accuracy, comparative study, patient outcomes, and accuracy were analyzed. We analyzed the studies based on the chosen papers, taking into account the accuracy, success rate, comparison studies, and patient outcomes.
RESULTS AND DISCUSSION
Our search identified 1,875 articles after applying the inclusion criteria for a scoping review. Subsequently, we excluded certain articles based on data items and outcomes, resulting in the inclusion of 24 studies; additionally, some other studies were manually added to our selection [Figure 1].
Figure 1. Flowchart for the updated PRISMA[46]. PRISMA: Preferred Reporting Items for Systematic Review and Meta-Analyses.
Here, we have mentioned the different applications of autonomous endoscopes and highlighted their advantages in terms of efficiency, accuracy, and improved patient outcomes. This section is organized based on the types of endoscopes and their areas of application.
AI in endoscopy
Esophagogastroduodenoscopy
Esophagogastroduodenoscopy (EGD) is used for the detection of upper gastrointestinal lesions. One of the fatal malignancies in the GI tract is esophageal adenocarcinoma, and the precursor lesion of this malignancy is Barrett’s esophagus (BE). Increasing dysplasia grades are the strongest indicator of how this malignancy will proceed. Currently, virtual chemoendoscopy and systematic 4-quadrant biopsies are most frequently used to identify visible lesions, followed by systematic 4-quadrant biopsies for the remainder of the BE segment. Recent research showed that this method’s miss rate is 25%, sampling mistakes occur, and it cannot identify below-surface epithelium.
In order to improve dysplasia diagnosis in BE, an AI system called VLE (volumetric laser endomicroscopy) and an artificial intelligence algorithm named IRIS (Intelligent Real-time Image Segmentation) were created [Table 2][10]. The esophageal wall may be seen in great detail in cross-section due to an advanced endoscopic imaging tool. However, deciphering the 1,200 pictures produced by VLE scans can be difficult. IRIS was created to help endoscopists by automatically emphasizing characteristics linked to dysplasia, such as abnormal epithelial glands, layer loss, and increased surface signal intensity. The findings demonstrated that there was no discernible difference between the two groups in the overall interpretation time. The IRIS-VLE group’s unenhanced VLE interpretation time, however, was drastically shortened. All endoscopically non-visible dysplastic regions were also found by IRIS-enhanced VLE, which greatly reduced the time required for later interpretation of images obtained without IRIS[10].
AI in endoscopy
AI system | Application | Key finding |
VLE & IRIS[10] | Barrett’s dysplasia | Improved dysplasia diagnosis, reduced interpretation time |
ENDOANGEL[11,12] | Gastric polyps & Cancer detection | High accuracy, improved anesthesia quality, fewer blind spots |
EAIRS[13] | Esophagogastroduodenoscopy | 95.2% accuracy, better lesion detection than endoscopists |
SSD-GPNet[15] | Gastric polyp detection | Real-time detection at 50fps, improved |
WLE vs. AI[16] | Gastric neoplasm detection | AI reduces neoplasm miss rate |
CADe[18] | Colonoscopy | Higher detection rate for small and proliferative polyps |
AI for LNM[23] | T1 colorectal cancer | 100% sensitivity, specificity; reduces unnecessary surgery |
CCE AI[24] | Incomplete colon capsule detection | Enhanced localization, identification of incomplete OC |
Magnetically Guided soft capsule endoscope for wireless biopsy[25] | Capsule endoscopy | Promising method for safe and targeted biopsy capabilities |
WCC[9] | Colon screening | Non-invasive, limitations in mobility and control |
The “ENDOANGEL”, a new AI system, was developed to improve the early detection of gastric polyps and gastric cancer[11]. The system was developed utilizing deep convolutional neural networks and reinforcement learning with the objectives of detecting early gastric cancer lesions and monitoring blind spots (gastric regions ignored during EGD). ENDOANGEL had considerably fewer blind spots but took more time for inspection than the control group in a trial with 1,050 patients who were randomly allocated to 498 ENDOANGEL patients and 504 control patients. The accuracy of the ENDOANGEL group’s real-time stomach cancer identification was 84.7% per lesion, 100% sensitivity, and 84.3% specificity after they found 196 gastric lesions with pathological data[11]. ENDOANGEL also significantly improves anesthesia quality during gastrointestinal endoscopy with shorter emergence and recovery times, higher satisfaction scores, and fewer overall adverse events[12].
The Endoscopic automatic image reporting system (EAIRS) is an intelligent system for automatic photo documentation during esophagogastroduodenoscopy. It was tested with 210,198 images and outperformed endoscopists with an accuracy of 95.2% with higher rates of completeness in capturing anatomical landmarks and detecting lesions[13]. Another study compared the endoscopic gastric cancer detection rate between expert endoscopists and AI[14]. Results showed that AI diagnosed 100% of patients, while expert endoscopists diagnosed only 94.2% of patients. The per-image rate of gastric cancer diagnosis was higher in AI, with an 11.8% difference[14].
A study presented Single Shot MultiBox Detector (SSD) for Gastric Polyps (SSD-GPNet), a convolutional neural network for real-time polyp detection based on the SSD architecture[15]. The enhanced SSD achieves real-time polyp detection with 50 frames per second and improves mean average precision from 88.5% to 90.4% with minimal time-performance loss. The system also improves polyp detection recalls by over 10%, particularly in small polyp detection, helping endoscopists find missed polyps and decreasing gastric polyp miss rates. This technology may be useful in daily clinical practice to reduce physician burden[15].
White light endoscopy (WLE) is the first-line tool for the detection of gastric neoplasms. A study compared WLE with AI-assisted endoscopy[16]. They evaluated the impact of AI on detecting focal lesions, diagnosing gastric neoplasms, and reducing the missed rate. The AI-first group had a significantly lower gastric neoplasm miss rate. AI-assisted endoscopy has the potential to improve detection of gastric neoplasms[16]. Another study investigated the prospective of multispectral narrow-band imaging (mNBI) for automated, quantitative detection of oropharyngeal carcinoma (OPC). Results showed that mNBI achieved higher accuracy in classifying tumors compared to WLE. This automated clinical detection of OPC could improve surgical visualization, enable early diagnosis, and facilitate high-throughput screening[17].
Colonoscopy
Colonoscopy is used for the detection of lesions in the colon and rectum. It is considered the gold standard for reducing the morbidity and mortality associated with colorectal cancer by detecting and removing precancerous lesions. About 80%-90% of colorectal polyps evolve from adenomatous polyps[18]. If left unattended, these polyps may undergo malignant transformation, resulting in colorectal cancer. The main factors that affect detection rates are blind areas and human errors. Indeed, even the course of a single workday can have a significant impact on the adenoma detection rate of endoscopists[19].
To address this challenge, a system called computer-aided detection (CADe) has been introduced. This works by obtaining colonoscopy videos and using automatic image extraction and machine learning-based AI algorithms to evaluate the CADe system’s performance. It has been proven that CADe detection rate is higher for detecting small and proliferative polyps. Hence, this system is used as a feasible tool for the detection of polyps and adenomas[20]. A CADe system called Endoscreener developed by Shanghai Wision AI, China, concluded that when compared to high-definition white-light colonoscopy, there was a rise in first-pass adenoma per colonoscopy and a decrease in the rate of adenoma misses and sessile serrated lesions[21]. Thus, the CADe system can be beneficial in improving polyp detection during colonoscopies[20].
The level of AI assistance may also vary between different centers, influenced by factors such as endoscopist gender, insertion time, withdrawal time, patient’s age, and waist circumference[22]. When the withdrawal time is longer, the insertion time is shorter and the patients are elderly, the polyp detection rate is higher due to the longer time of inspection and higher incidence of polyps. Finally, AI-assisted colonoscopy has great potential in detecting overlooked, small, or proliferative polyps. However, operating standards should be developed to optimize its performance[23].
Moreover, beyond identifying precancerous tumors, AI can also help identify lymph node metastasis (LNM) and determine if further surgery is necessary and foretell the presence of LNM in patients with T1 colorectal cancer[23]. The AI model examined clinicopathological parameters and predicted whether LNM was positive or negative. The gold standard for LNM presence was operative specimens. When compared against American, European, and Japanese criteria, the model demonstrated 100% sensitivity, specificity, and accuracy. The study indicated that AI greatly decreased needless extra surgery following T1 colorectal cancer endoscopic resection without missing LNM positive[23].
Capsule endoscopy
In contemporary colonoscopy practice, another modality gaining interest is Colon capsule endoscopy (CCE) or optical colonoscopy (OC). Large-colon screening and improved knowledge of bowel cancer symptoms have resulted in a rise in OC demand. CCE appears to be a potential approach for reducing these needs. After an incomplete OC, CCE investigations are possible. Nonetheless, due to the high proportion of inadequate tests, the diffusion of CCE in clinical practice is delayed. Patients frequently favor CCE due to its low complication rate. An AI-based system was created to enhance capsule localization, allowing the identification of incomplete CCE investigations that overlap with incomplete OC[24].
Capsule endoscopy shows promise as a minimally invasive diagnostic and therapeutic tool for the gastrointestinal tract. However, the current clinical application of wireless capsules is limited to passive monitoring due to the lack of control over the position of the capsules. To overcome this limitation, researchers are working on magnetically guided and actuated capsule robots for the next generation of endoscopy. The study introduces a new wireless biopsy method using a magnetically actuated soft capsule endoscope that carries and releases thermo-sensitive microgrippers inside the stomach to obtain tissue samples. The method shows promise in ex vivo experiments. Existing biopsy mechanisms have limitations, such as the inability to approach targeted tissue, limited visibility, and low success rates. The proposed method combines a centimeter-scale untethered capsule with magnetic actuation, enabling advanced functions and offering safe and targeted biopsy capabilities in capsule endoscopy[25].
The wireless colon capsule (WCC) has evolved as a non-invasive alternative screening approach, although it has significant drawbacks. The operation is expensive, needs more thorough colon preparation, and can be difficult to swallow. The capsule’s mobility is regulated by peristalsis and cannot be accurately controlled to investigate a specific area of the colonic mucosa. Despite these limitations, WCC technology is frequently employed in various medical locations worldwide because of poor patient acceptability of standard colonoscopy, restricted availability, long waiting lists, and limited staff. Endorobots for colonoscopy are being developed to address these issues and improve the patient experience overall[9].
Autonomous robotic endoscopes
Bronchoscopy
Lung cancer is the leading cause of death regardless of gender. Bronchoscopy is the traditional method used to detect and perform a biopsy of the lung lesion. The bronchial tree within the lung is less elastic than the hollow viscous structures of the gastrointestinal tract, which are more difficult to navigate autonomously because of an increased risk of perforation. Consequently, the development of autonomous navigational bronchoscopy has progressed more smoothly, with several autonomous robots already available on the market. These technologies are typically used to perform biopsies on lung lesions that are difficult to reach by traditional bronchoscopy[26]. Among these innovations is Ares, a teleoperated endoluminal device created by Auris Surgical Robotics, designed to enhance visibility in the respiratory tract during bronchoscopy [Table 3]. It uses a primary and operative system with a power box, control modules, and a twin-arm robot with six degrees of freedom. The primary surgeon console allows for haptic interaction and tactile force feedback. Ares has Food and Drug Administration (FDA) certification for lung disease treatment and diagnosis. Johnson & Johnson acquired Ares in 2019[27].
Robotically-assisted flexible endoluminal and endovascular systems
Endoscopic robotic system | Description | Key features | Approvals and certifications |
PMAR needle equipment | Developed by the University of Genoa | Needlescopic diagnosis | Small robot dimensions |
Enos single access robotic surgical system | Developed by Titan Medical | Single port orifice robotic technology | 3D and 2D high-definition cameras |
Sensei X robotic catheter system | Developed by Hansen Medical Inc. | Primary and operative system for cardiac catheter manipulation | FDA-approved for the treatment and diagnostics of lung disorders |
Vascular catheter CorPath GRX | Developed by Corindus Vascular Robotics (acquired by Siemens Healthineers) | Trajectory control of coronary guide wires | Allows operators to carry out procedures from a radiation-protected workstation |
Aeon phocus cardiac catheter | Developed by Aeon Scientific | Remote control of interventional instruments using magnetic fields | High accuracy and improved safety |
ARES robotic endoscopy system | Developed by Auris Surgical Robotics | Teleoperated endoluminal system for bronchoscopy | FDA-approved for the treatment and diagnostics of lung disorders |
EndoQuest Robotics | Developed by EndoQuest Robotics | Teleoperated endoluminal system | Console system enabling single incision and endoluminal Gastrointestinal procedures, not FDA-approved |
A patient-specific soft magnetic catheter with a diameter of 2 mm can travel autonomously, allowing it to penetrate deeper into parts of the anatomy inaccessible to normal apparatus. The catheter has the same diameter as the rigid instruments but is soft, anatomy-specific, completely shape-forming, and robotically controlled remotely. Compared to tip- and axially magnetized catheters, the design method displayed enhanced navigation in terms of interaction with the environment and reduced aiming error. The study showed up to a 50% increase in accurate tracking, a 50% reduction in obstacle contact time during navigation, and a 90% improvement in aiming error over the state-of-the-art. The suggested method has the potential to improve navigational bronchoscopy by decreasing the requirement for longer learning curves for the equipment[26].
Deep learning models have been effective for lung lesions, such as Deep Q reinforcement learning (RL), an unsupervised deep learning (DL) algorithm, which is used for autonomous endoscope navigation, overcoming problems in robotic healthcare caused by heart and lung movement. The researchers wanted to use deep Q RL within a CNN (Convolutional Neural Network), a.k.a, DQNN (Deep Q Neural Network), to make the first endoscopic tasks autonomous. This method allows the bronchoscope to move safely based on pictures obtained during bronchoscopy, improving navigation accuracy. Q-learning RL selects the best course of action for various scenarios by balancing exploration and exploitation. The algorithm tolerates greater mistakes during exploration for increased learning and better future actions, providing more accurate and autonomous medical operations[28].
Becoming skilled in maneuvering and effectively communicating with surgeons demands significant training. The natural hand tremors we all have can lead to shaky views, and holding the endoscope steady during long procedures can be tiring. These challenges fade away with robots, which not only outperform humans in precision, but also do not get tired. An example of this is the Automated Endoscopic System for Optimal Positioning (AESOP®, Computer Motion, Inc., Goleta, CA, USA)[8]. AESOP pioneered in this field through gestures, switches, and voice commands. This robot utilized a 3-degree-of-freedom SCARA - type structure, which enhances stability during procedures. AI robotics has tremendous potential to support health professionals in their daily tasks, particularly through deep learning methods such as deep neural networks, which have exhibited remarkable success in surpassing human performance. However, the complexity of these high-dimensional and non-linear models poses a challenge as they become less interpretable by human experts[29].
Robotic endoscopes have the potential to improve endoscopic procedures, but present efforts are hindered by movement and visual issues. One study used endoscopic forceps, an auto-feeding mechanism, and positional feedback to perform autonomous intervention procedures on a Robotic Endoscope Platform (REP). For tool position estimation, the workspace model and Structure from Motion technique are employed, and a visual system for regulating REP position and forceps extension is established. The results reveal a 5.5% accuracy and a 43% success rate for 1-cm polyps, a 67% success rate for 2-cm polyps, and an 81% success rate for 3-cm polyps. This is the first attempt at automating colonoscopy intervention onboard a mobile robot, and these approaches are applicable to in vivo devices[30]. A workspace model for tool position estimation that employs Structure from Motion (SfM) to estimate target-polyp positions was developed for pioneering automated colonoscopy interventions on a mobile robot, with potential applicability in future endoscopic devices for in vivo use. This visual system controls REP’s position and forceps extension, achieving accurate results across different anatomical environments. The technique demonstrates success rates of 43%, 67%, and 81% for polyps of varying sizes[30].
The integration of robots with rigid endoscopes has led to transformative advancements in medical technology. Another milestone was the TISKA Endoarm, which has a specialized mechanism ensuring precision. ViKY, the ViKY robotic scope holder, allowed control through pedals or voice, earning CE marking and FDA approval[31]. Systems like SOLOASSIST™ and AutoLap™ enabled voice and image-guided control, while EndoAssist™, Navio™, and CUHK endoscope manipulator expanded options. Interface diversity, including gaze control, voice, and joystick, enriches practical utility. The amalgamation of robotics and endoscopy has reshaped the landscape of minimally invasive medical procedures[8].
Passive navigation: magnetic flexible endoscopy
Both active and passive manipulation techniques have propelled the evolution of flexible endoscopy. The magnetic flexible endoscope (MFE) has been developed for painless colonoscopy, which could provide a major improvement and disruption in the early detection and treatment of colorectal diseases and relies on passive navigation as visual images from the tip of the endoscope are used to enable a robotic arm and magnet to passively drag the scope through the colon[16]. This method is relevant to a variety of additional endoscopic applications where the environment is unstructured and provides major navigational issues[32].
MFE has acquired popularity in medical endoscopy; however, advancement has mostly focused on navigation because of the tip’s instability. To offer stability during interventional movements, a novel model-based Linear Parameter Varying (LPV) control technique is developed. The linearization of the dynamic interaction between the external actuation system and the endoscope ensures global stability and resilience to external disturbances. In a benchtop colon simulator, the LPV methodology outperforms an intelligent teleoperation control method on the MFE platform, lowering mean orientation error by 45.8%[23]. However, due to control issues, converting magnetically actuated endoscopes for clinical usage has failed. Advanced control techniques capable of helping and providing a simple user interface with shortened process durations would enable magnetic colonoscopy to be clinically translated, broadening and enhancing patient care. Although navigation with magnetic endoscopes has been shown for stomach screening, catheter guiding, and bronchoscopy, navigation in mobile and complicated settings such as the colon requires more advanced control[32]. MFEs show progress in navigation, but interventional tasks such as biopsies face control challenges, which some authors have proposed overcoming with Endorobots[33].
Endorobots
Despite much advancement in endoscopes, only a few endorobotic devices have been given clearance. Some of those are Aer-o-Scope GI-View, NeoGuide Endoscopy System, ColonoSight, and Invendoscope™ Medical devices. They showcase a range of propulsion mechanisms, operational functionalities, and working channels, highlighting the evolving landscape of endorobot technology in the field of colonoscopy[9]. Another type of endorobot is the Autonomous microrobotic endoscopy system for navigating the human colon. Its components are vision-guided microrobot, pneumatic locomotion, and real-time image-based guidance. With the potential for adaptive exploration and enhanced procedural safety, this system shows a promising future for revolutionizing endoscopic procedures[34]. A major drawback of this technology is that it is not cost-effective, which is not anticipated to change in the foreseeable future.
Active navigation
In an effort to maintain stability and control at the tip of endoscopes, more actively controlled endoscopes have been developed. The EndoDrive (ECE Medical Products, Erlangen, Germany) offers electro-mechanical insertion of flexible endoscopes, with shaft positioning and driving controlled through a foot pedal, freeing both hands for tip manipulation. The NeoGuide™ Endoscopy System (NES) integrates multiple electromechanically controlled segments, allowing the system to adapt to the colon’s shape during insertion. The Endotics® Endoscopy System (EES) employs pneumatically-driven components to simulate caterpillar-like movement for locomotion. Meanwhile, the Invendoscope™ (Invendo Medical, Kissing, Germany) is a motor-controlled colonoscope with wheels for propulsion. The Aer-O-Scope™ (GI View Ltd., Ramat Gan, Israel) utilizes pneumatics to drive two balloons for active locomotion[8,35]. Expertise also plays a crucial role in flexible robotic technology, particularly in endovascular procedures for successfully navigating target lesions and treating arterial lesions using the vascular control catheter[36]. These active approaches in flexible endoscopy play a pivotal role in enabling efficient colon exploration and interventions[8,34].
DISCUSSION
The diagnostic process in endoscopy is inherently subjective and depends heavily on the expertise of the operator, resulting in significant variability. There exists a pressing imperative to enhance the quality and consistency of endoscopic examinations. The integration of AI in the creation of autonomous endoscopic systems holds the potential to elevate the standard of diagnostic and therapeutic instruments, establish uniformity in procedural protocols, and improve the cognitive and physical well-being of healthcare practitioners.
FE has inherent limitations such as complexity, patient pain, and a lack of intuitiveness, needing highly skilled staff and a lengthy and costly training procedure. Overcoming these limitations would allow screening procedures such as colonoscopies to become ubiquitous and significantly impact the early detection of malignant diseases. Robots used in colonoscopy show advanced computer-integrated intelligent systems for achieving the following objectives[35]:
1. Alleviating the safety and efficiency of conventional healthcare practices in diagnosis and treatment, encompassing aspects such as accuracy, efficacy, safety, and dependability.
2. Alleviating the daily workload through enhanced ergonomic design, contributing to improved comfort for operators.
3. Amplifying the intervention capabilities of endoscopists and standardizing their procedural proficiency, even during remote operations.
4. Amplifying the scope of feasible medical interventions, thereby expanding the potential realm of procedures.
Currently, enhanced autonomy in medical robotics refers to the progressive development of robotic systems, with six levels of autonomy defined by increased intelligence [Table 4][37,38]. Level 5 fully autonomous robots have elements of deep learning, Machine learning, Computer vision, and natural language processing. The robots that encompass all these elements are considered strong AI. However, as of now, such robots have not been developed. Instead, we currently have Weak AI robots capable of independently performing tasks like automatic linear stapled gastrointestinal anastomosis and aligning robotic arms during the tilting of the operating table[39]. Several ways to distinguish between weak and powerful AI by observing the robot can be seen in Table 5[39].
Levels of Endoscopic Autonomy[38]
Level 0 | No Autonomy | Robotically controlled endoscopes follow commands given by humans |
Level 1 | Robot assistance | A robotically controlled endoscope provides mechanical assistance and guidance during a task while the human retains continuous control |
Level 2 | Task autonomy | Robotically-controlled endoscopes are autonomous in tasks started by humans |
Level 3 | Conditional autonomy | Robotically-controlled endoscopes generate task strategies, but require human input to select or approve them, allowing them to perform tasks with reduced oversight |
Level 4 | High autonomy | Robotically controlled endoscopes have the capability to make medical decisions but still operate under the supervision of a qualified healthcare worker |
Level 5 | Full autonomy | Robotically controlled endoscopes do all the work without humans |
How to distinguish between weak and powerful AI by observing the robot[40]
Aspect | Weak AI (Reactive agents) | Strong AI (Deliberative agents) |
Definition | Automatic actions | Has information gathering, knowledge improvement, exploration, learning, and full autonomy |
Complexity | Simple tasks | Understands complex problems with advanced solutions equal to or superior to humans |
Intelligence | Lacks the intelligence | Complex interpretation of problems and advanced problem-solving capabilities |
Examples | Autonomous stapling, robotic arm alignment during table tilting | Full autonomy, decision-making based on extensive knowledge and understanding |
Cognitive abilities | Lacks cognitive abilities like desires, beliefs, and intentions | May have cognitive abilities like desires, beliefs, and intentions in the future |
Current state | Exists and is demonstrated in certain autonomous actions | Not yet in surgical robots, remains a long-term goal |
Progression towards strong AI | Represents initial steps towards more advanced AI | Represents the eventual evolution and maturity of AI |
Autonomy levels | Typically falls in levels 0-3 of surgical autonomy | Corresponds to levels 4-5 of surgical autonomy, showing higher levels of independent decision-making |
As more autonomous devices are developed, there is a need to reevaluate the present Risk Levels framework for analyzing emerging technology. To appropriately assess these improvements, a larger range of risk categories should be defined by creating Risk Level 0 to encourage the development of more complex surgical equipment, notably those falling within Levels 2 and 3 of surgical autonomy, and Risk Level 5 to advance devices with surgical autonomy at Levels 4 or 5. Furthermore, the development of robots such as the STAR (Surgical Training and Autonomous Robot) adds an intriguing dimension to the future landscape[40]. It raises the question of whether these robots will primarily serve as supplements to tele-manipulation or if they will revolutionize non-console robotic surgery by keeping both the surgeon and the robot at the patient’s bedside. Although the immediate integration of autonomous robots into both situations is still possible, establishing the best long-term trajectory requires surgeons, endoscopists, and interventionalists to have a thorough grasp of AI and robotics. By doing so, we may take responsibility for the safe incorporation of autonomous surgical activities, avoiding undue industry influence and protecting patient well-being[41].
As mentioned, magnetically actuated endoscopes have shown promise in terms of reducing discomfort, lowering costs, improving diagnostic capabilities, and improving therapeutic treatments. However, they are hampered by poor control at their tip, mainly limited to diagnosis, and may require endorobots to perform therapeutic interventions. Human-robot cooperation navigation strategy for soft endoscope intervention, they autonomously detect cavity centers and adjust endoscope orientation. This strategy improves efficiency and safety in procedures. This innovation holds a promising future for reducing endoscopist workload and enhancing the automation of interventions[5]. Robotic navigation system (RNS) for paroxysmal atrial fibrillation, the RNS showed similar success rates to manual ablation, and lower power settings led to better outcomes over time. Increased experience with the robotic system results in shorter procedure times, higher success rates, and improved outcomes and efficiency in atrial fibrillation management[42]. A novel three-linked-sphere robot created through 3D printing was developed to reinforce learning for autonomous navigation in tight spaces. This shows the ability to navigate frictional surfaces and achieve notable efficiency, about 90%, compared to non-learning counterparts and for diverse applications, offering scalability and compactness[43].
Explainable artificial intelligence (XAI), which addresses the interpretability of black-box techniques, is required in the field of health due to the necessity of explainability and transparency. XAI is becoming more and more important as the standards for medical AI solutions improve, necessitating the understanding of machine decision-making by human professionals. Uncertainty surrounds the cost-effectiveness of a new XAI robot-assisted cystoscopy technique that shows promise for the detection of both cancer and benign lesions. Cystoscopy, the Gold Standard for detecting bladder cancer, has difficulty categorizing a wide range of results, which may result in false negatives and positives. Further study and advancements in optical techniques may improve detection rates and treatment outcomes in cystoscopy, whereas robotic-assisted cystoscopy powered by XAI has the ability to minimize these issues and boost diagnostic accuracy[29].
Currently, when used in minimally invasive settings, autonomous robotic surgery shows potential for improving effectiveness and safety in soft tissue surgery. The robotic endoscopic small bowel anastomosis used in this study in phantom and real intestinal tissues serves to illustrate an improved autonomous technique for minimally invasive soft tissue surgery. Through the use of the developed technology, surgeons can select from a set of surgical plans that the robot has generated on its own. Results demonstrate increased accuracy and consistency compared to manual and robot-assisted approaches, demonstrating the potential of high-level autonomous surgical robots to enhance patient outcomes and provide access to standardized surgical techniques. However, difficulties still exist, particularly in contexts with a wide range of variables and constrained access, such as laparoscopic procedures. Anastomosis is an important soft tissue surgery procedure that calls for careful consideration of a number of criteria, including tissue health, technique, materials, and surgeon[37].
The innovative robotic flexible endoscope system intended for minimally invasive bariatric surgery (LBS) was another type of robot utilized in laparoscopy. They created the UR5 robot and a flexible endoscope with a one-of-a-kind “reinforced double helix continuum mechanism” joint that is dependent on image-based visual serving. This enhances both rigidity under pressure and torsional strength compared to a simpler helix structure. The effectiveness of the visual serving control method can be enhanced by incorporating deep learning techniques to increase its efficiency and viability[44]. A robotic system called autonomous laparoscopic robotic suturing system outperformed manual suturing by improving consistency in suture spacing 2.9 times and eliminating the need for repositioning and adjustments. This system was developed to address challenges posed by limited space during minimally invasive surgery and may prove feasible during other endoscopic procedures[45].
LIMITATIONS
In this research, we have incorporated articles that demonstrated superior outcomes. Studies with insignificant results and those with balanced percentages were excluded to provide a clearer summary of the data. Nonetheless, it should be noted that there are also studies that did not yield significant results.
CONCLUSION
AI and robotic technology have made major advances in endoscopic techniques. AI technologies are suggestive of improving diagnosis in dysplasia via EGD, greatly reducing interpretation time, increasing identification of stomach polyps and cancer, and enhancing identification of non-visible dysplastic areas, as well as major progress in anesthetic quality during endoscopy. Colonoscopy-based AI techniques have also shown assurance in enhancing polyp and adenoma detection rates, potentially lowering missed diagnoses. Additionally, AI can anticipate lymph node metastases, minimizing the need for unneeded procedures. Capsule endoscopy, mainly wireless and AI-based techniques, is emerging as a non-invasive diagnostic tool with the prospective to transform gastrointestinal medicine. These current emerging techniques give emphasis on transforming medicine towards more safe, efficient, and autonomous endoscopic treatments; however, further standardization and clinical validation are required for wider implementation.
DECLARATIONS
Authors’ contributions
Drafting of the manuscript, editing of the manuscript, technical support, administrative support: Boini A
Editing of manuscript, administrative support: Acciufi S
Conceptualization: Croner R
Technical Support, administrative support : Milone L, Illanes A, Turner B
Conceptualization, drafting of the manuscript, editing of the manuscript, technical support, administrative support: Gumbs AA
Availability of data and materials
Upon reasonable request.
Financial support and sponsorship
None.
Conflicts of interest
Gumbs AA is the CEO of Talos Surgical; Turner B is the Chairman of Talos Surgical; Milone L is the Chief Medical Officer of Talos Surgical. Other authors declared that there are no conflicts of interest.
Ethical approval and consent to participate
Not applicable.
Consent for publication
Not applicable.
Copyrights
© The Author(s) 2023.
REFERENCES
1. Alkire BC, Raykar NP, Shrime MG, et al. Global access to surgical care: a modelling study. Lancet Glob Health 2015;3:e316-23.
2. Chahal D, Byrne MF. A primer on artificial intelligence and its application to endoscopy. Gastrointest Endosc 2020;92:813-20.e4.
3. Ferlay J, Colombet M, Soerjomataram I, et al. Cancer statistics for the year 2020: an overview. Int J Cancer 2021;149:778-89.
4. World Health Organization. The true death toll of COVID-19: Estimating global excess mortality. Available from: https://www.who.int/data/stories/the-true-death-toll-of-covid-19-estimating-global-excess-mortality. [Last accessed on 7 Dec 2023].
5. Jiang W, Zhou Y, Wang C, Peng L, Yang Y, Liu H. Navigation strategy for robotic soft endoscope intervention. Int J Med Robot 2020;16:e2056.
6. Gumbs AA, Abu-Hilal M, Tsai TJ, Starker L, Chouillard E, Croner R. Keeping surgeons in the loop: are handheld robotics the best path towards more autonomous actions? (A comparison of complete vs. handheld robotic hepatectomy for colorectal liver metastases). Art Int Surg 2021;1:38-51.
7. Lu Y, Wei R, Li B, et al. Autonomous intelligent navigation for flexible endoscopy using monocular depth guidance and 3-D shape planning. arXiv 2023:In press.
9. Manfredi L. Endorobots for colonoscopy: design challenges and available technologies. Front Robot AI 2021;8:705454.
10. Kahn A, McKinley MJ, Stewart M, et al. Artificial intelligence-enhanced volumetric laser endomicroscopy improves dysplasia detection in Barrett’s esophagus in a randomized cross-over study. Sci Rep 2022;12:16314.
11. Wu L, He X, Liu M, et al. Evaluation of the effects of an artificial intelligence system on endoscopy quality and preliminary testing of its performance in detecting early gastric cancer: a randomized controlled trial. Endoscopy 2021;53:1199-207.
12. Xu C, Zhu Y, Wu L, et al. Evaluating the effect of an artificial intelligence system on the anesthesia quality control during gastrointestinal endoscopy with sedation: a randomized controlled trial. BMC Anesthesiol 2022;22:313.
13. Dong Z, Wu L, Mu G, et al. A deep learning-based system for real-time image reporting during esophagogastroduodenoscopy: a multicenter study. Endoscopy 2022;54:771-7.
14. Niikura R, Aoki T, Shichijo S, et al. Artificial intelligence versus expert endoscopists for diagnosis of gastric cancer in patients who have undergone upper gastrointestinal endoscopy. Endoscopy 2022;54:780-4.
15. Zhang X, Chen F, Yu T, et al. Real-time gastric polyp detection using convolutional neural networks. PloS One 2019;14:e0214133.
16. Wu L, Shang R, Sharma P, et al. Effect of a deep learning-based system on the miss rate of gastric neoplasms during upper gastrointestinal endoscopy: a single-centre, tandem, randomised controlled trial. Lancet Gastroenterol Hepatol 2021;6:700-8.
17. Mascharak S, Baird BJ, Holsinger FC. Detecting oropharyngeal carcinoma using multispectral, narrow-band imaging and machine learning. Laryngoscope 2018;128:2514-20.
18. Shakir T, Kader R, Bhan C, Chand M. AI in colonoscopy - detection and characterisation of malignant polyps. Art Int Surg 2023;3:186-94.
19. Marcondes FO, Gourevitch RA, Schoen RE, Crockett SD, Morris M, Mehrotra A. Adenoma detection rate falls at the end of the day in a large multi-site sample. Dig Dis Sci 2018;63:856-9.
20. Liu WN, Zhang YY, Bian XQ, et al. Study on detection rate of polyps and adenomas in artificial-intelligence-aided colonoscopy. Saudi J Gastroenterol 2020;26:13-9.
21. Glissen Brown JR, Mansour NM, Wang P, et al. Deep learning computer-aided polyp detection reduces adenoma miss rate: a united states multi-center randomized tandem colonoscopy study (CADeT-CS Trial). Clin Gastroenterol Hepatol 2022;20:1499-507.e4.
22. Xu L, He X, Zhou J, et al. Artificial intelligence-assisted colonoscopy: a prospective, multicenter, randomized controlled trial of polyp detection. Cancer Med 2021;10:7184-93.
23. Ichimasa K, Kudo SE, Mori Y, et al. Artificial intelligence may help in predicting the need for additional surgery after endoscopic resection of T1 colorectal cancer. Endoscopy 2018;50:230-40.
24. Deding U, Herp J, Havshoei AL, et al. Colon capsule endoscopy versus CT colonography after incomplete colonoscopy. Application of artificial intelligence algorithms to identify complete colonic investigations. United Eur Gastroent 2020;8:782-9.
25. Yim S, Gultepe E, Gracias DH, Sitti M. Biopsy using a magnetic capsule endoscope carrying, releasing, and retrieving untethered microgrippers. IEEE Trans Bio-Med Eng 2013;61:513-21.
26. Pittiglio G, Lloyd P, da Veiga T, et al. Patient-specific magnetic catheters for atraumatic autonomous endoscopy. Soft Robot 2022;9:1120-33.
27. Cepolina F, Razzoli RP. An introductory review of robotically assisted surgical systems. Int J Med Robot 2022;18:e2409.
28. Gumbs AA, Grasso V, Bourdel N, et al. The advances in computer vision that are enabling more autonomous actions in surgery: a systematic review of the literature. Sensors 2022;22:4918.
29. O’Sullivan S, Janssen M, Holzinger A, et al. Explainable artificial intelligence (XAI): closing the gap between image analysis and navigation in complex invasive diagnostic procedures. World J Urol 2022;40:1125-34.
30. Zhang Q, Prendergast JM, Formosa GA, Fulton MJ, Rentschler ME. Enabling autonomous colonoscopy intervention using a robotic endoscope platform. IEEE Trans Bio-Med Eng 2020;68:1957-68.
31. Gumbs AA, Crovari F, Vidal C, Henri P, Gayet B. Modified robotic lightweight endoscope (ViKY) validation in vivo in a porcine model. Surg Innov 2007;14:261-4.
32. Martin JW, Scaglioni B, Norton JC, et al. Enabling the future of colonoscopy with intelligent and autonomous magnetic manipulation. Nat Mach Intell 2020;2:595-606.
33. Barducci L, Scaglioni B, Martin J, Obstein KL, Valdastri P. Active stabilization of interventional tasks utilizing a magnetically manipulated endoscope. Front Robot AI 2022;9:854081.
34. Asari VK, Kumar S, Kassim IM. A fully autonomous microrobotic endoscopy system. J Intell Robot Syst 2000;28:325-41.
35. Ciuti G, Skonieczna-Żydecka K, Marlicz W, et al. Frontiers of robotic colonoscopy: a comprehensive review of robotic colonoscopes and technologies. J Clin Med 2020;9:1648.
36. Bismuth J, Duran C, Stankovic M, Gersak B, Lumsden AB. A first-in-man study of the role of flexible robotics in overcoming navigation challenges in the iliofemoral arteries. J Vasc Surg 2013;57:14S-9S.
37. Saeidi H, Opfermann JD, Kam M, et al. Autonomous robotic laparoscopic surgery for intestinal anastomosis. Sci Robot 2022;7:eabj2908.
38. Yang GZ, Cambias J, Cleary K, et al. Medical robotics - Regulatory, ethical, and legal considerations for increasing levels of autonomy. Sci Robot 2017;2:eaam8638.
39. Gumbs AA, Frigerio I, Spolverato G, et al. Artificial intelligence surgery: how do we get to autonomous actions in surgery? Sensors 2021;21:5526.
40. Leonard S, Wu KL, Kim Y, Krieger A, Kim PCW. Smart tissue anastomosis robot (STAR): a vision-guided robotics system for laparoscopic suturing. IEEE Trans Biomed Eng 2014;61:1305-17.
41. Gumbs AA, Gayet B. Why Artificial Intelligence Surgery (AIS) is better than current Robotic-Assisted Surgery (RAS). Art Int Surg 2022;2:207-12.
42. Rillig A, Lin T, Schmidt B, et al. Experience matters: long-term results of pulmonary vein isolation using a robotic navigation system for the treatment of paroxysmal atrial fibrillation. Clin Res Cardiol 2016;105:106-16.
43. Elder B, Zou Z, Ghosh S, et al. A 3D-printed self-learning three-linked-sphere robot for autonomous confined-space navigation. Adv Intell Syst 2021;3:2100039.
44. Zhang X, Li W, Ng WY, et al. An autonomous robotic flexible endoscope system with a DNA-inspired continuum mechanism. In: 2021 IEEE International Conference on Robotics and Automation (ICRA); 2021 May 30 - Jun 05; Xi’an, China. IEEE; 2021. pp. 12055-60.
45. Saeidi H, Le HND, Opfermann JD. Autonomous laparoscopic robotic suturing with a novel actuated suturing tool and 3D endoscope. In: 2019 International Conference on Robotics and Automation (ICRA); 2019 May 20-24; Montreal, Canada. IEEE; 2019. pp. 1541-7.
Cite This Article
Export citation file: BibTeX | RIS
OAE Style
Boini A, Acciuffi S, Croner R, Illanes A, Milone L, Turner B, Gumbs AA. Scoping review: autonomous endoscopic navigation. Art Int Surg 2023;3:233-48. http://dx.doi.org/10.20517/ais.2023.36
AMA Style
Boini A, Acciuffi S, Croner R, Illanes A, Milone L, Turner B, Gumbs AA. Scoping review: autonomous endoscopic navigation. Artificial Intelligence Surgery. 2023; 3(4): 233-48. http://dx.doi.org/10.20517/ais.2023.36
Chicago/Turabian Style
Boini, Aishwarya, Sara Acciuffi, Roland Croner, Alfredo Illanes, Luca Milone, Bruce Turner, Andrew A. Gumbs. 2023. "Scoping review: autonomous endoscopic navigation" Artificial Intelligence Surgery. 3, no.4: 233-48. http://dx.doi.org/10.20517/ais.2023.36
ACS Style
Boini, A.; Acciuffi S.; Croner R.; Illanes A.; Milone L.; Turner B.; Gumbs AA. Scoping review: autonomous endoscopic navigation. Art. Int. Surg. 2023, 3, 233-48. http://dx.doi.org/10.20517/ais.2023.36
About This Article
Special Issue
Copyright
Data & Comments
Data
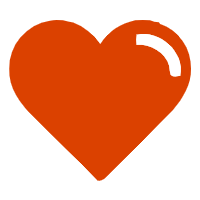

Comments
Comments must be written in English. Spam, offensive content, impersonation, and private information will not be permitted. If any comment is reported and identified as inappropriate content by OAE staff, the comment will be removed without notice. If you have any queries or need any help, please contact us at support@oaepublish.com.